ML enables early detection in EOL tests
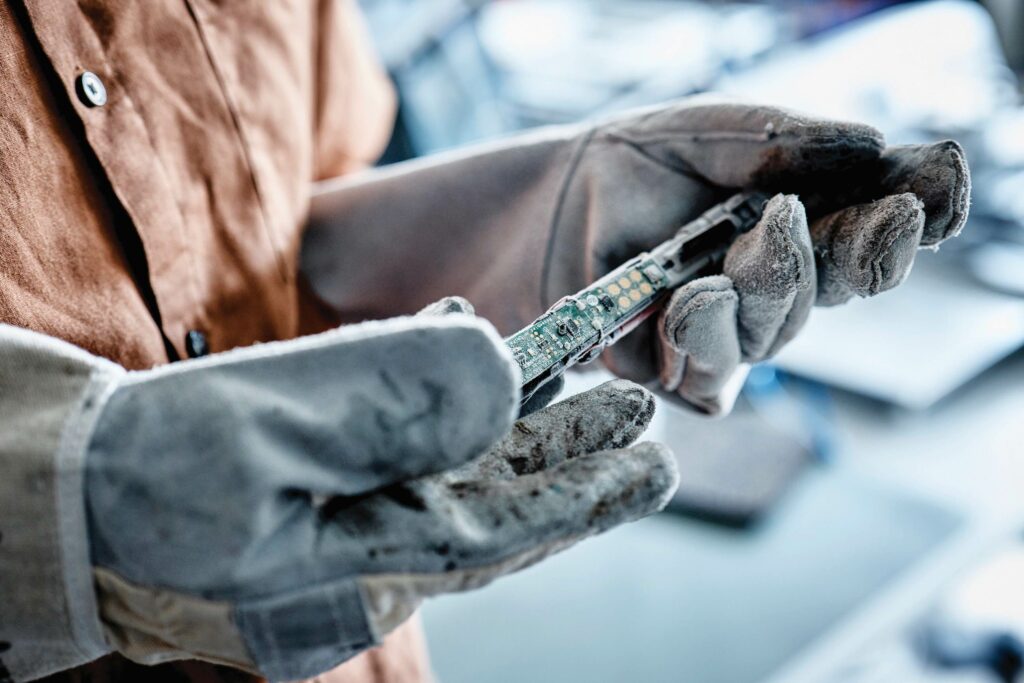
(Image courtesy of AITAD)
Machine learning (ML) with ultrasonic sensors is enabling early error detection during end-of-line (EOL) testing, writes Nick Flaherty.
AITAD in Germany has developed an embedded, AI ultrasonic sensor system that can detect significantly more faults in an e-mobility platform at an early stage of the production cycle.
During EOL testing of subcomponents in the automotive industry, various tests are carried out to prevent a faulty component being installed. For example, tests are carried out for production errors and completeness, from the tight fit of screws to the correct adjustment of a motor.
Classic EOL test procedures already use sensors, such as temperature, angle, vibration and pressure sensors for cold (externally moved), hot (self-moving) and performance tests in order to detect deviations from the ideal state of the component or subsystem.
Acoustic sensors are also used to search for deviating spectrum weightings in the data.
After final assembly in the end system, there is often a person who listens for component failures in the test operation, based on their years of experience. This is where the development of embedded AI sensors comes into play. With the AI and sensor fused together on one circuit board, data can be evaluated in real time in greater depth.
For example, an ultrasonic sensor that records signals at a sampling rate of hundreds of kilohertz and a vertical resolution of 32 bits generates several terabytes of raw data per day, even in small quantities. This volume of data could not practicably be transmitted via conventional network connections.
The embedded AI can assess the raw sensor data byte by byte to find anomalies. After evaluation, the data is deleted and the embedded AI module only passes on the information obtained from it. This makes the system more secure against data manipulation or theft.
To do this, the data must first be collected at the various test stations using acquisition hardware equipped with various types of sensor, correlated with the faults found by conventional means and transferred to a database.
A ML model is trained on this data to detect anomalies in the first step, but can later be adjusted more finely with different error types and localisation through classification.
“We were able to achieve a detection rate of over 95% early on in the EOL testing chain with a specially developed ultrasonic sensor solution at an automotive manufacturer, which in turn contributed to high savings,” said Viacheslav Gromov, founder and CEO of AITAD.
Higher error coverage is a result of the more complex pattern recognition and in-depth data evaluation of the ML model compared with classic sensor technology and algorithms with better screening rates by processing large amounts of data. There is no requirement for network infrastructure or ongoing cloud costs.
The system can minimise risk and damage, particularly in the event of supplier-parts changes, new production start-ups or assembly changes.
“Depending on the use case, an embedded AI ultrasonic sensor system can be developed, trained and integrated for as little as around 100,000 euros,” said Gromov.
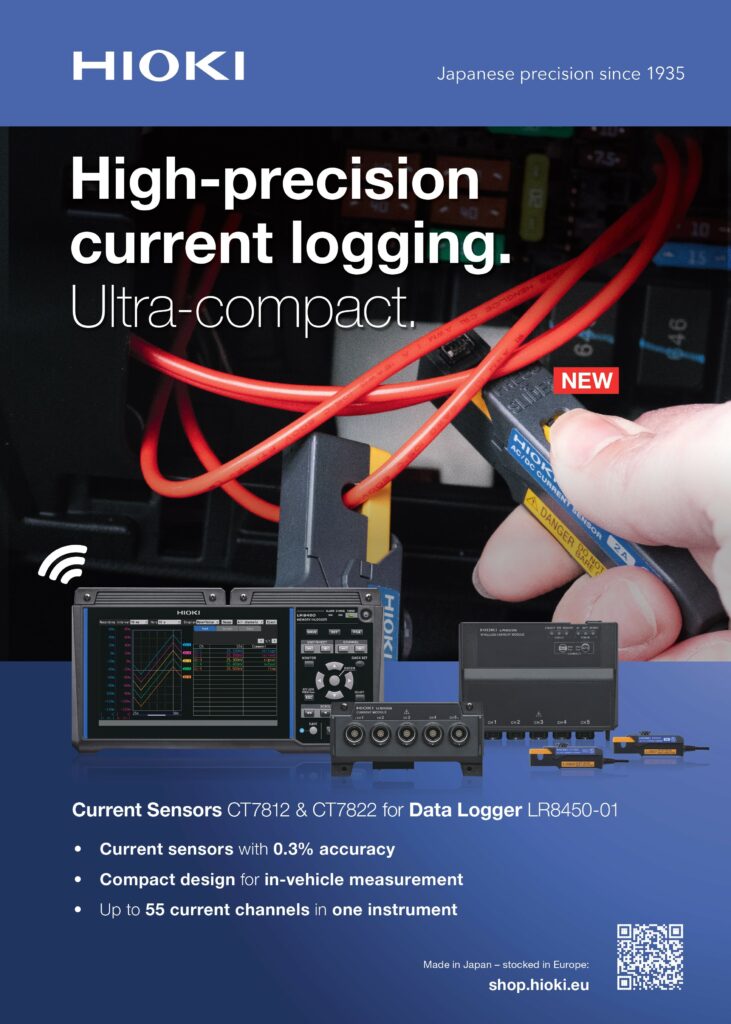
ONLINE PARTNERS
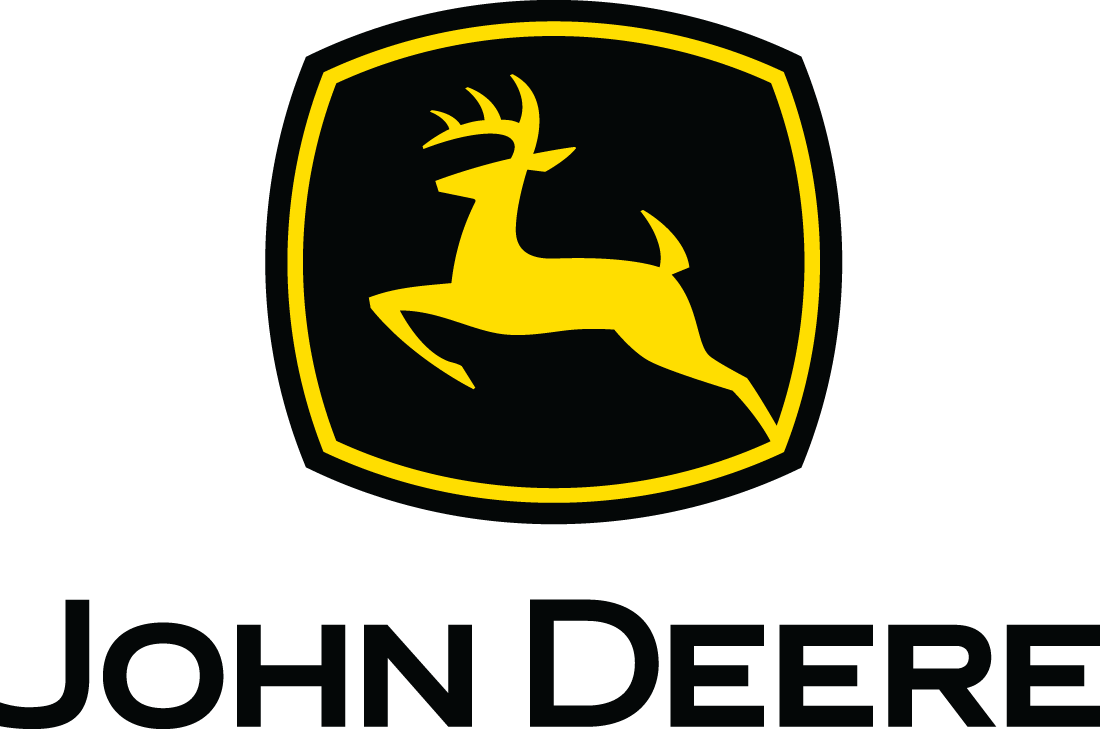
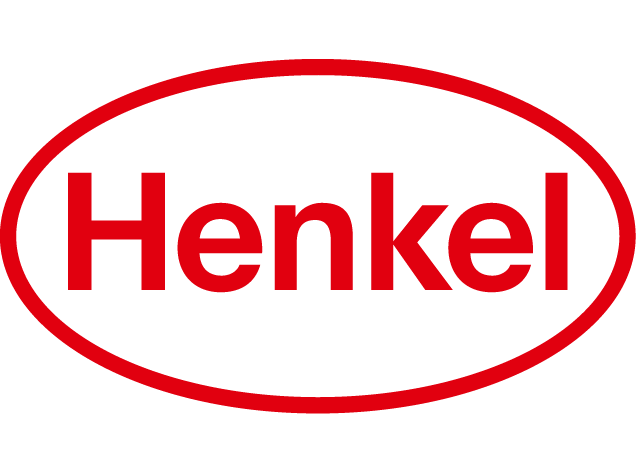
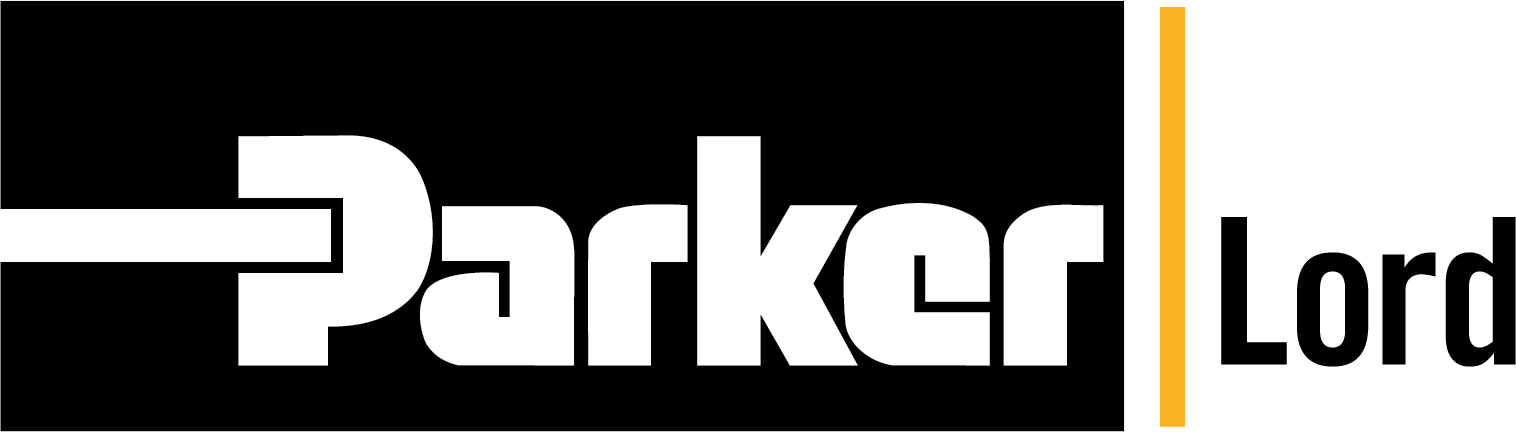
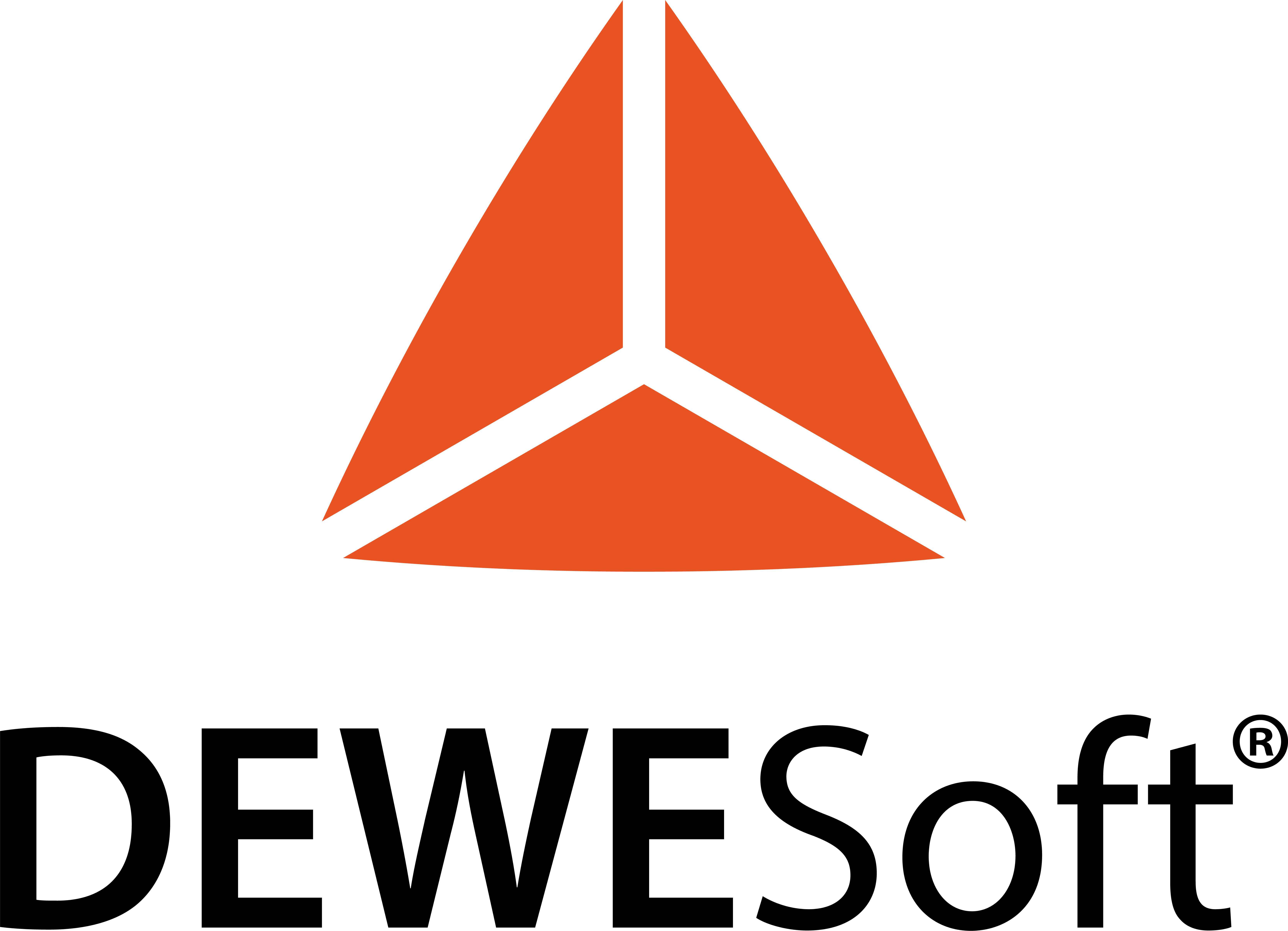
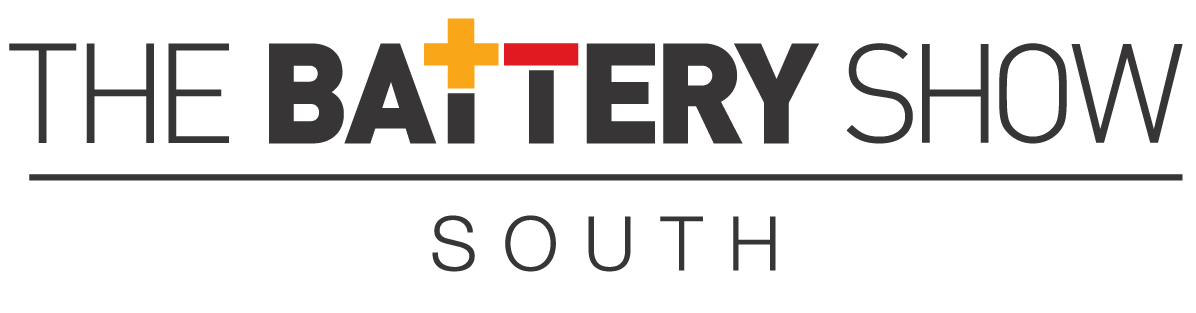
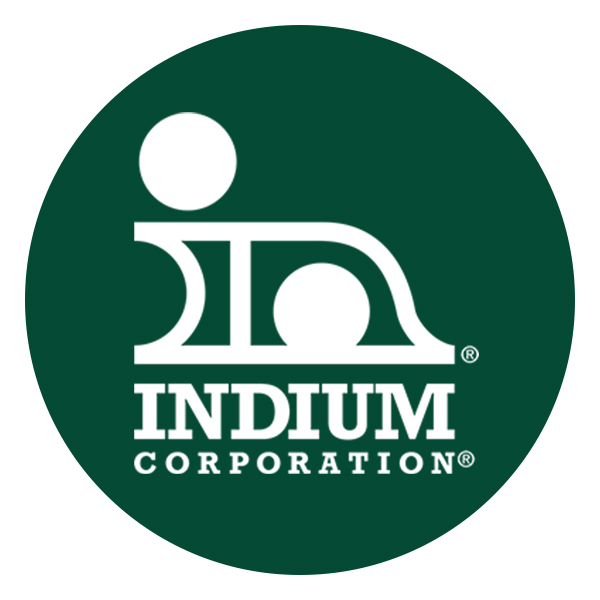

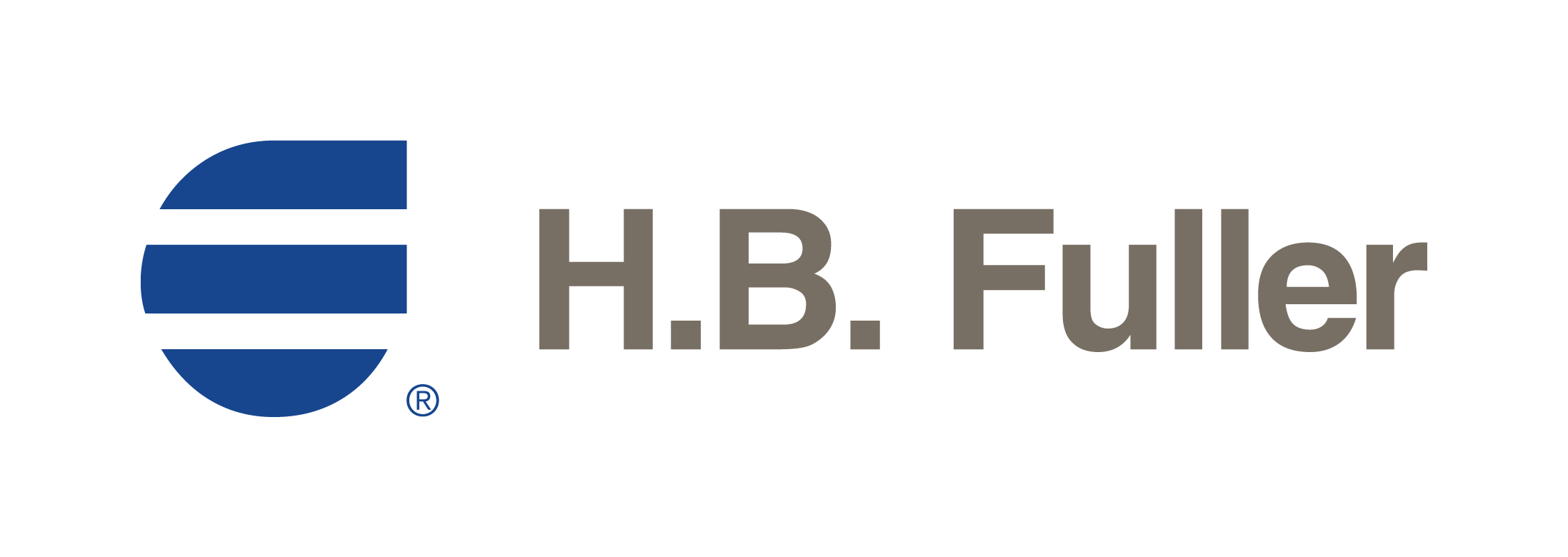
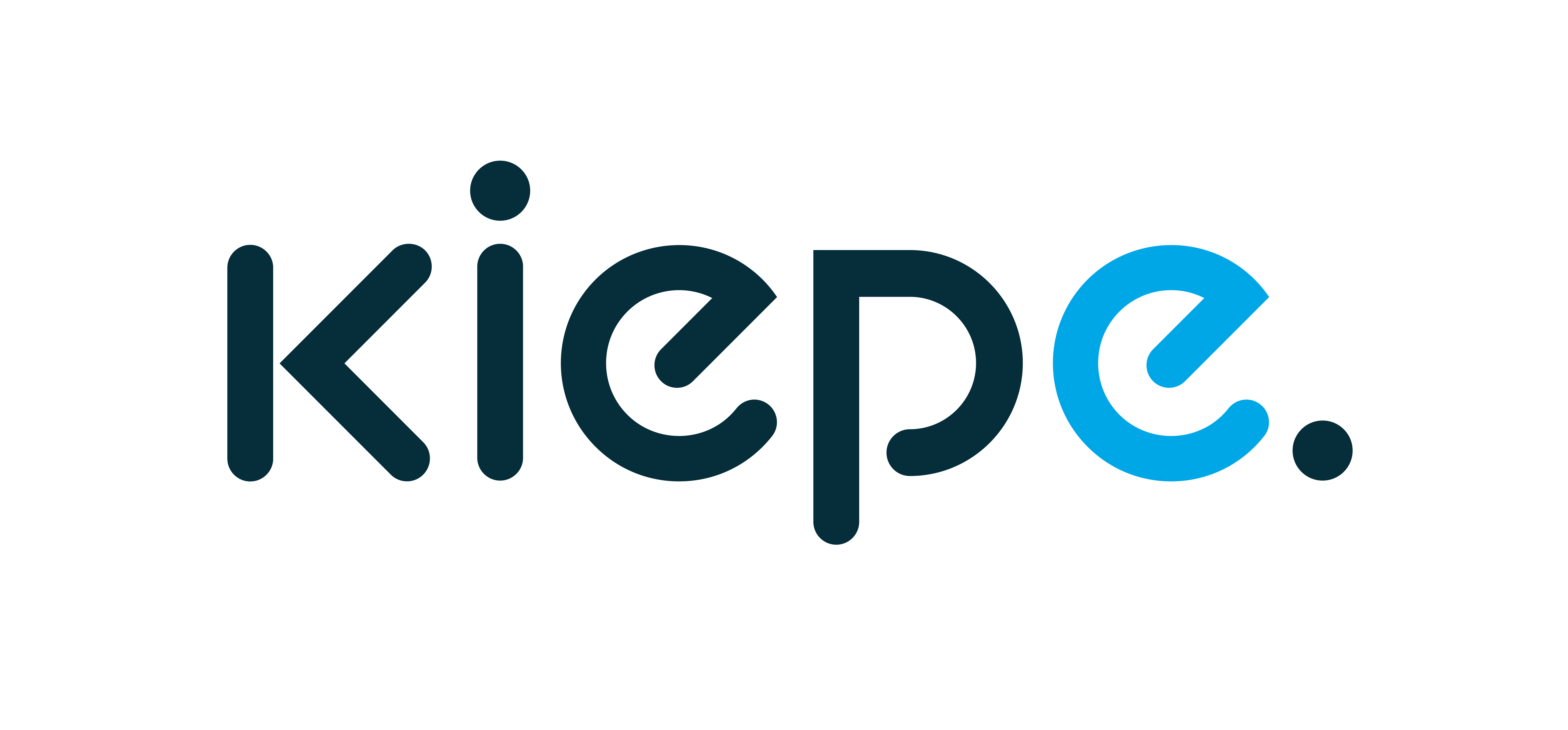
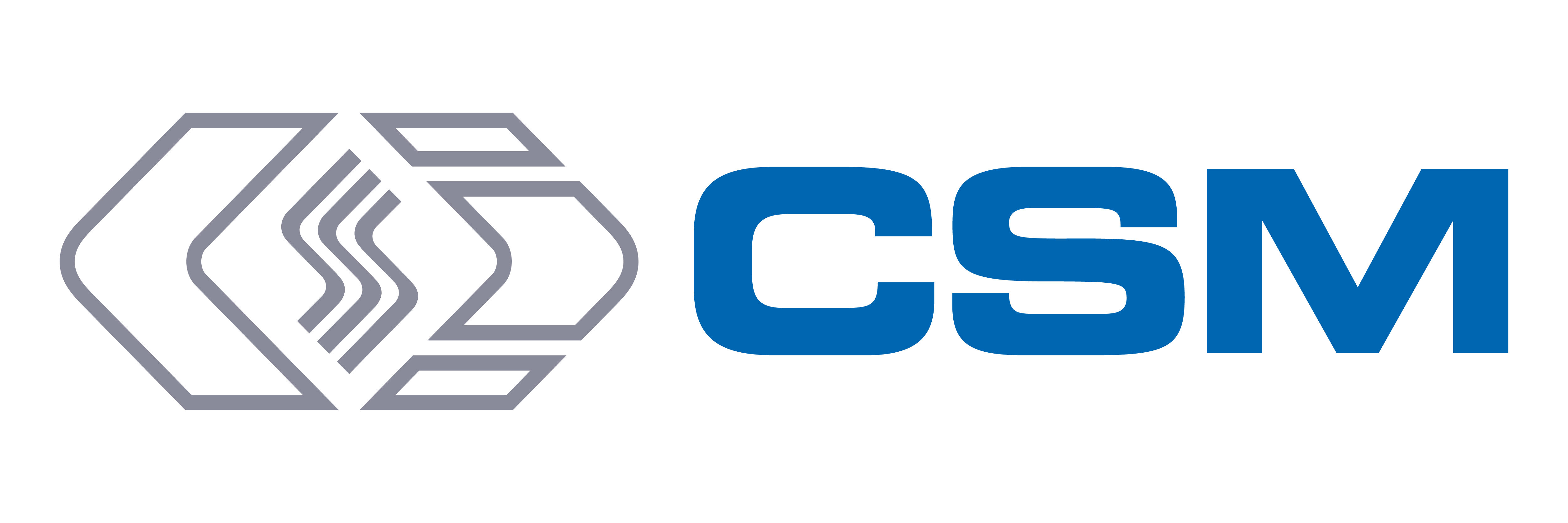