Cloud twin helps to extend EV range
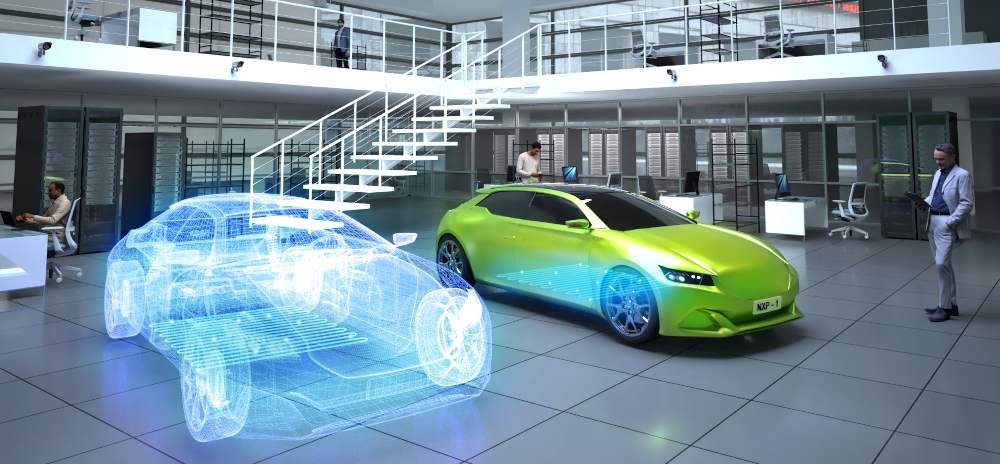
NXP has connected a battery management system (BMS) through its cloud computing gateway to create a digital twin of a vehicle battery (writes Nick Flaherty).
This enables machine learning on the data in the digital twin models in the cloud to improve the battery range by as much as 12%, as well as improving the efficiency, safety and lifetime of the battery pack.
The high-voltage battery management system (HVBMS) is based around the S32K3 microcontroller and sensors with a precision of 0.5% to extend battery life safely. Having precise measurements of the battery’s state of health and charge helps to optimise the charging and maximise the driving range with accurate diagnostics.
The HVBMS consists of a battery management unit, a cell monitoring unit (CMU) and a battery junction box. The CMU has four MC33775A analogue front ends in a daisy chain to support up to 56 cells with cell balancing of 300 mA.
The digital twin software, from Electra Vehicles, runs on the S32K3 with ARM Cortex-M7 controller cores operating in lockstep for ASIL-D functional safety certification. NXP has developed a new package for this controller, the MaxQFP, which reduces the package footprint by up to 55% compared with the standard quad flat pack (QFP) package.
Electra’s EVE-AI tool is used to build an adaptive digital twin of the battery cells on a vehicle. The digital twin processes the data to identify cycles in a time series and extracts features at both the battery and vehicle level.
The adaptive cell modelling system then dynamically selects the most appropriate model for a specific usage profile. This continuously updates cell modelling based on historical and real-time data to inform charge and discharge decisions.
The digital twin provide a range of features, from dynamic state of charge (SOC) limits that expand the minimum and maximum SOC during safe conditions to extend range without compromising the safety functions of the BMS, to providing velocity recommendations to the driver to maximise range, working with existing route planning software.
When the vehicle is charging, dynamic thermal charging adapts the temperature across the battery pack for fast charging with limited harm to cell lifetime. The digital twin also provides a predictive charging model that analyses the behaviour patterns of a particular user to customise the charging strategy, while a battery fault predictability function uses AI to classify battery a cell’s usable lifetime and predict unsafe cell conditions.
The BMS is then connected through NXP’s S32G GoldBox vehicle networking reference design to the cloud to share data from the digital twin, again using the EVE-AI technology.
The GoldBox is based on the S32G2 processor, which has four ARM Cortex-A53 cores with three ARM Cortex-M7 cores that operate in lockstep for real-time applications. This subsystem has a low-latency comms engine and a packet-forwarding engine to accelerate Ethernet data and the link to the cloud, and includes a hardware security engine for secure boot and accelerated security services to protect the data.
The cloud connectivity allows the AI model on the BMS to continuously learn from data collected from fleets of EVs around the world for faster and more precise adaptation to specific driver profiles.
Another potential application for the cloud connection is EV fleet management, providing fleet operators with usage insights such as vehicle charging times and battery predictive diagnostics. Battery care centres can also use this information to reduce downtimes with rapid diagnostics, and EV charging station operators can optimise their charging services.
ONLINE PARTNERS
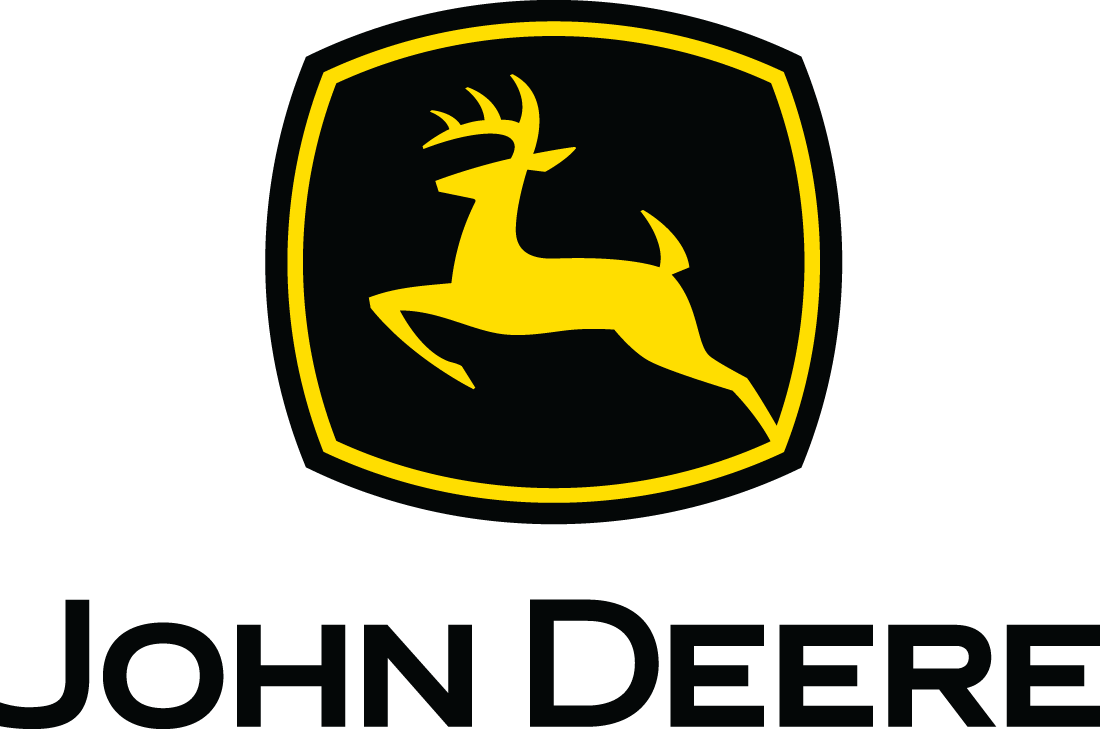
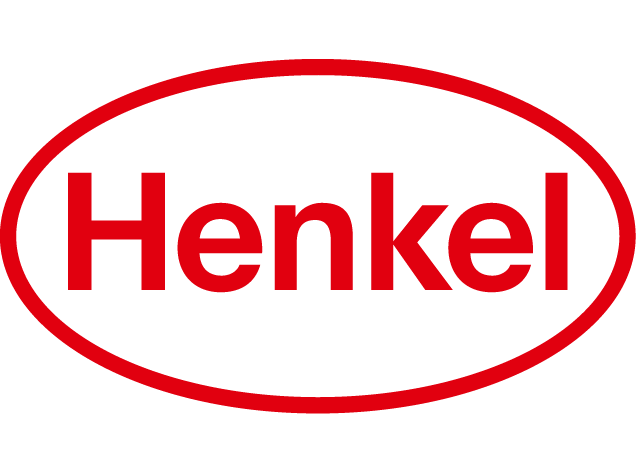
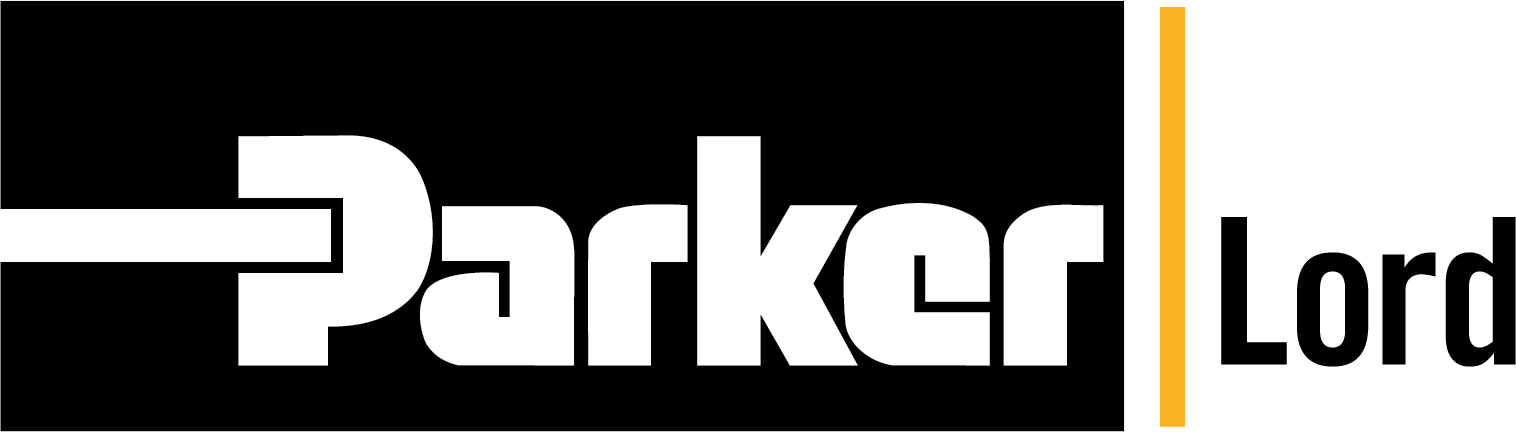
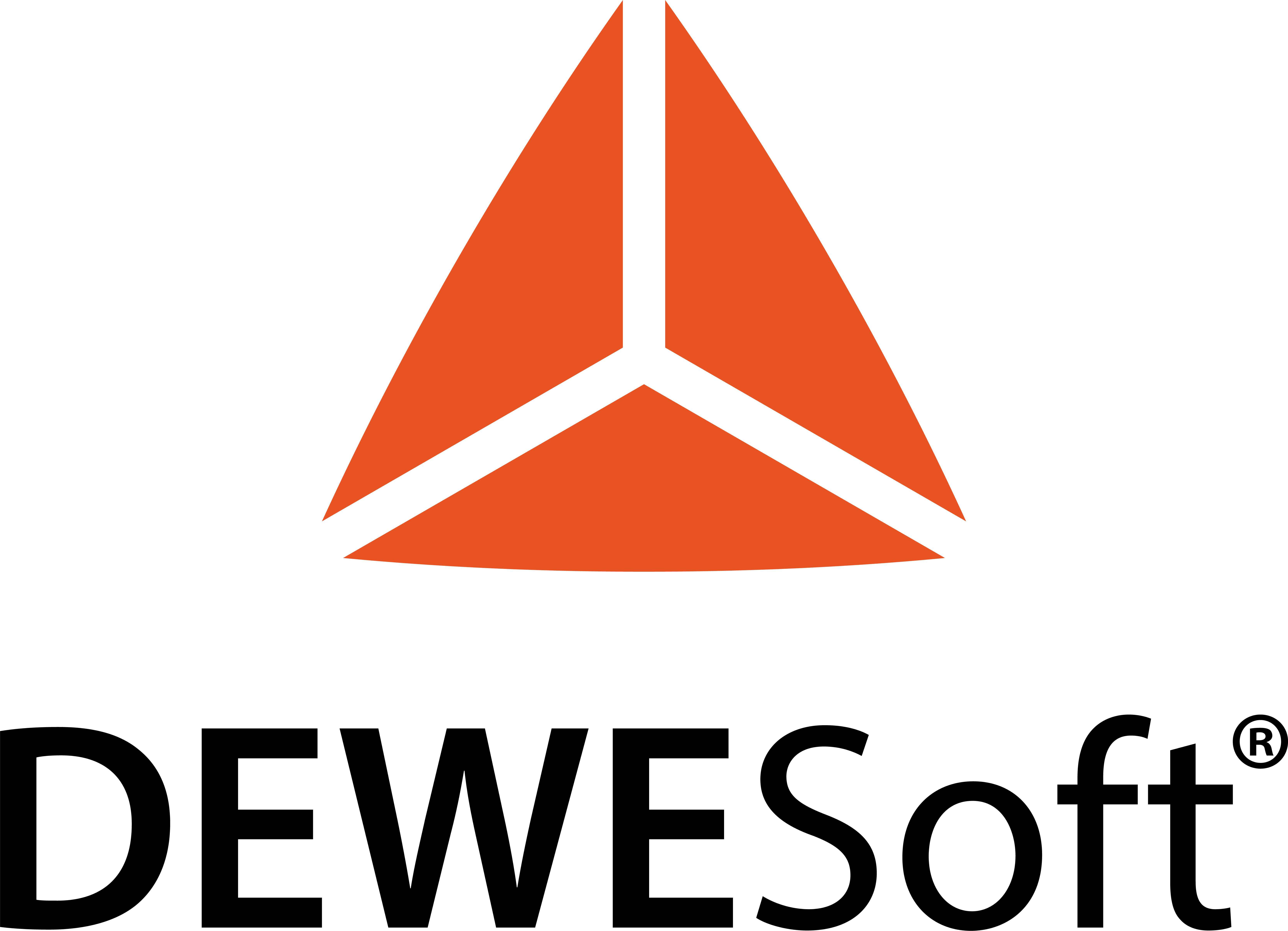
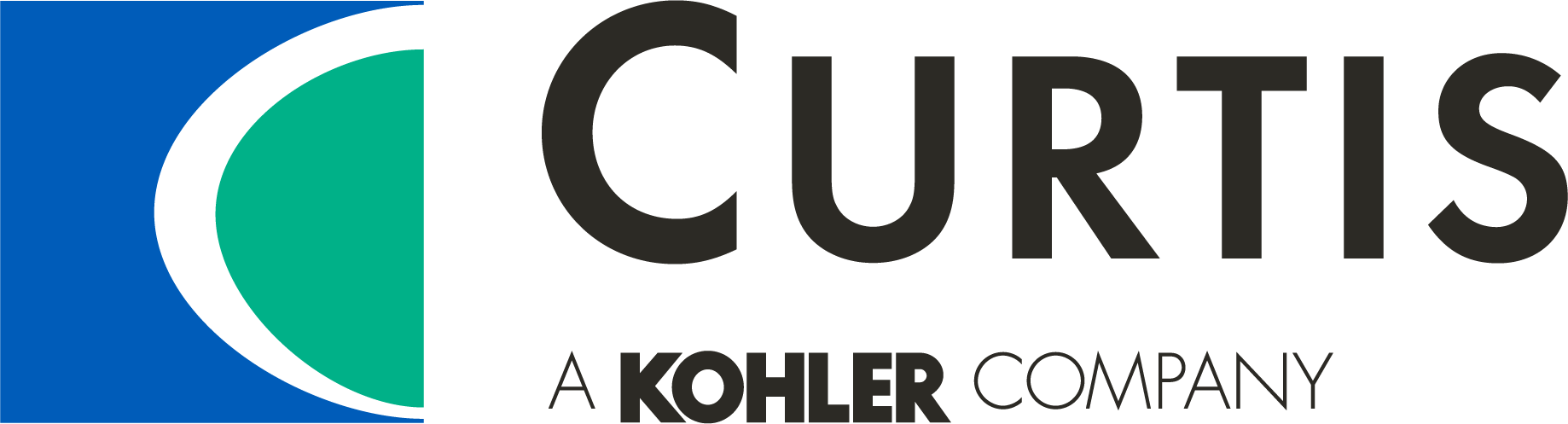
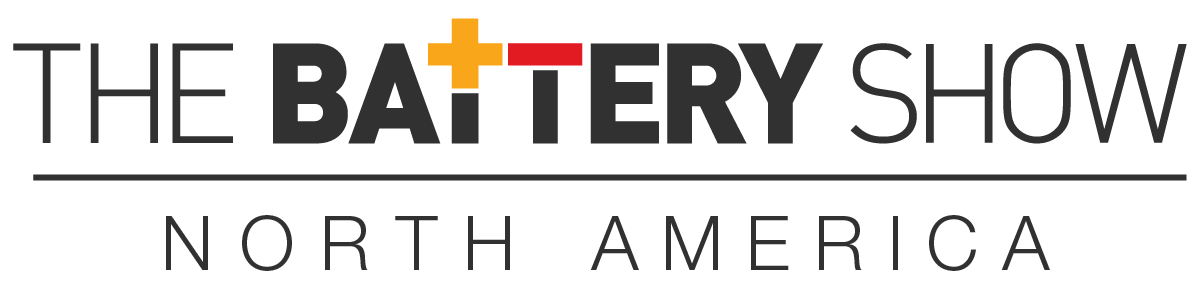
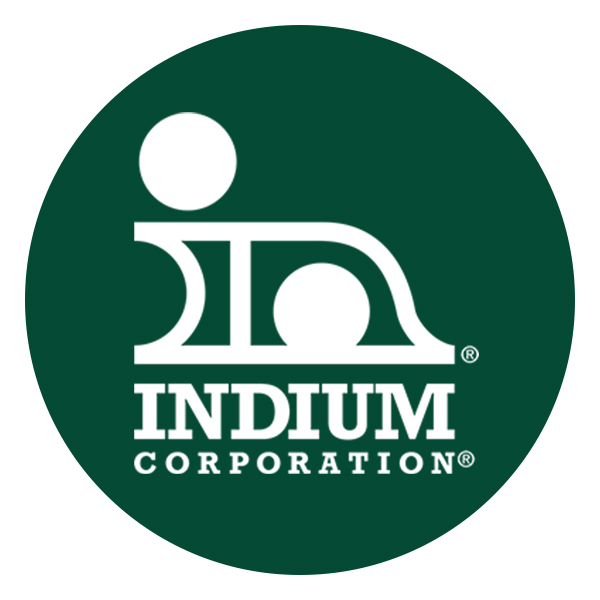

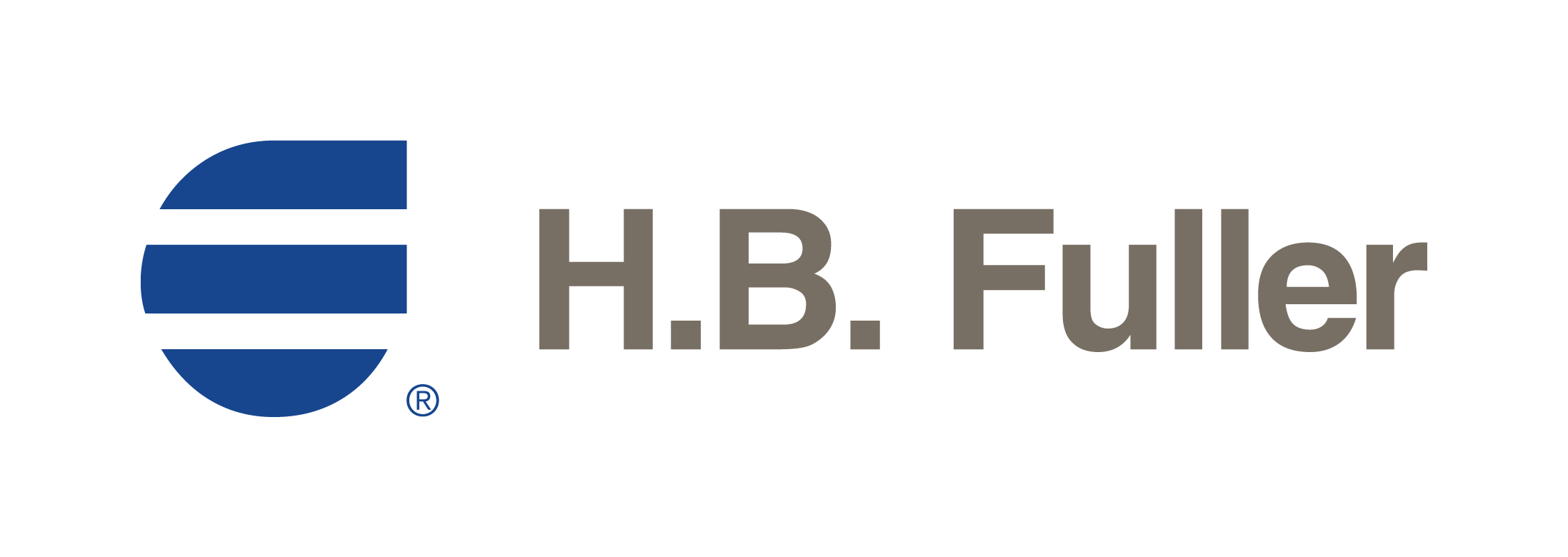
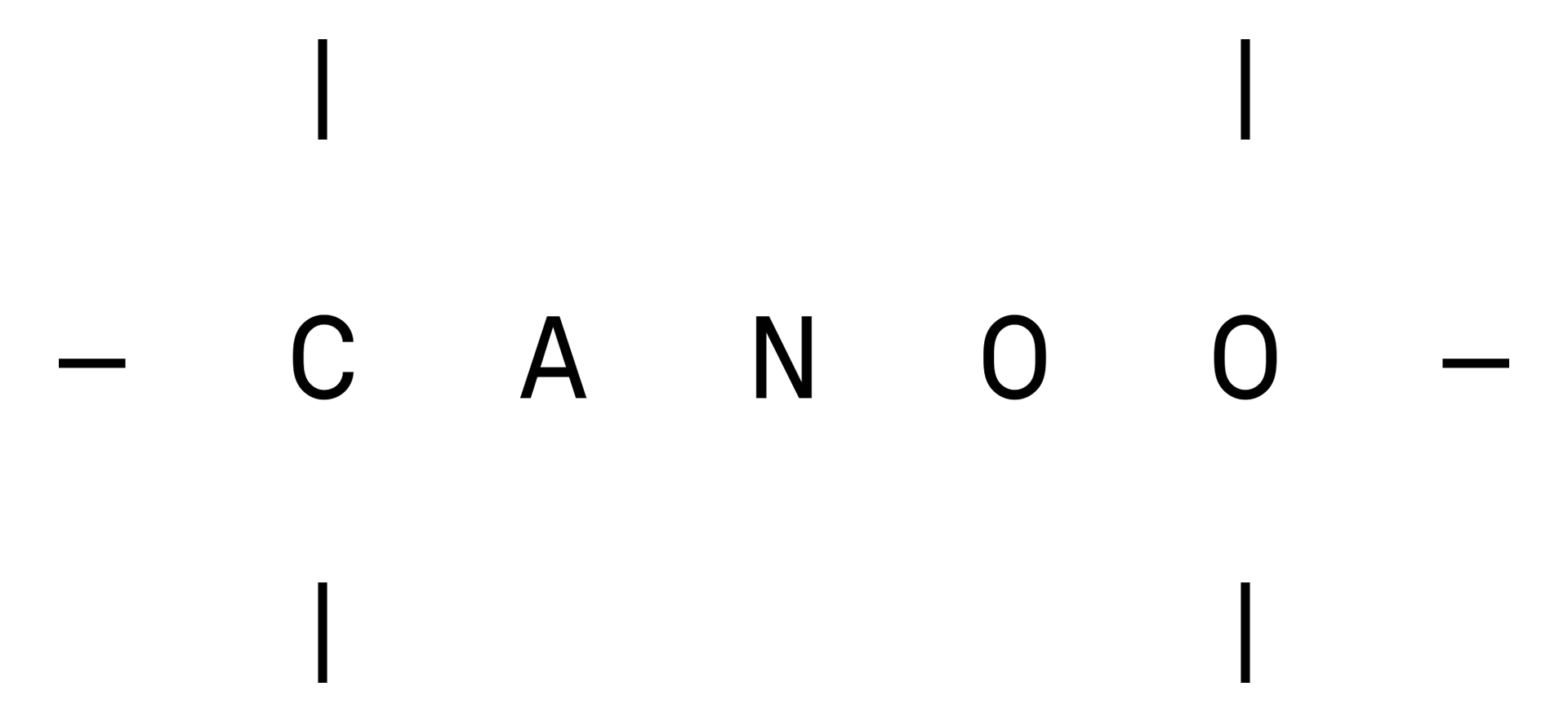