ChatGPT for battery chemicals
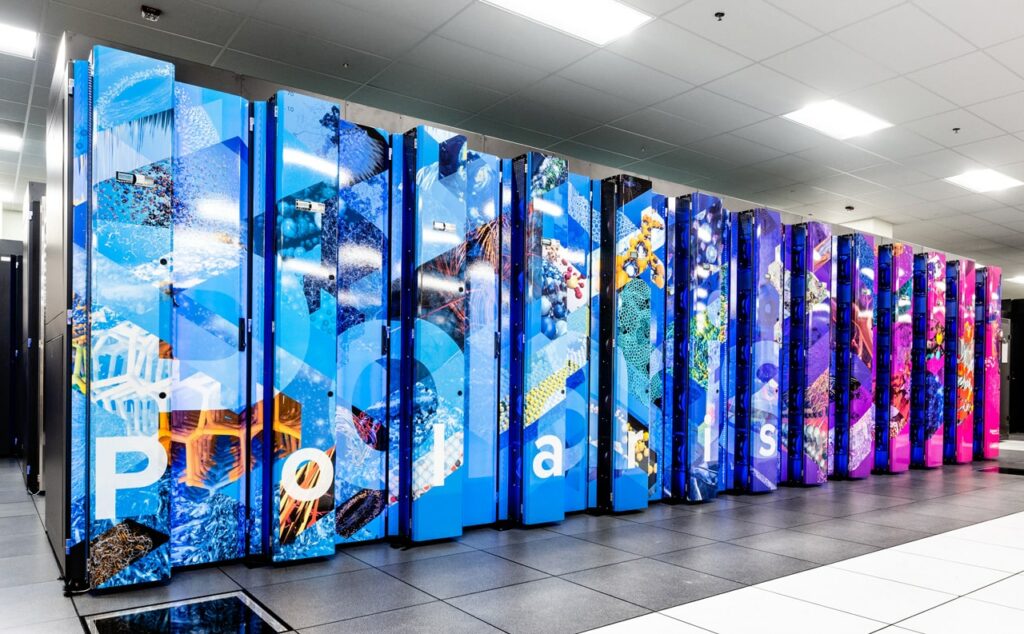
(Image courtesy of Argonne National Laboratory)
A team of researchers led by the University of Michigan in the US is developing a generative AI model to produce new molecules for batteries, writes Nick Flaherty. The machine learning framework will be developed using 200,000 node hours on the Polaris, a 34-petaflop supercomputer at Argonne National Laboratory. The team will build a foundational model for molecules, similar to the GPT models that support applications such as ChatGPT. The new model will focus on small organic molecules with relevance to batteries.
The chemical model will be fed text-based representations of atomic structures with no additional information like chemical properties. It will be used to predict better battery electrolytes.
When the model is able to predict missing atoms in small organic compounds, the team will move on to fine-tuning—feeding it the properties of some compounds and asking it to predict the properties of others. Through iterative feedback, they intend to build an AI that can master small organic molecule chemistry.
Once the model is up and running, the team will ask it to predict electrolytes suited to a particular pair of electrodes. It will then experimentally test each prescription in the lab with a robotic setup, Clio.
ONLINE PARTNERS
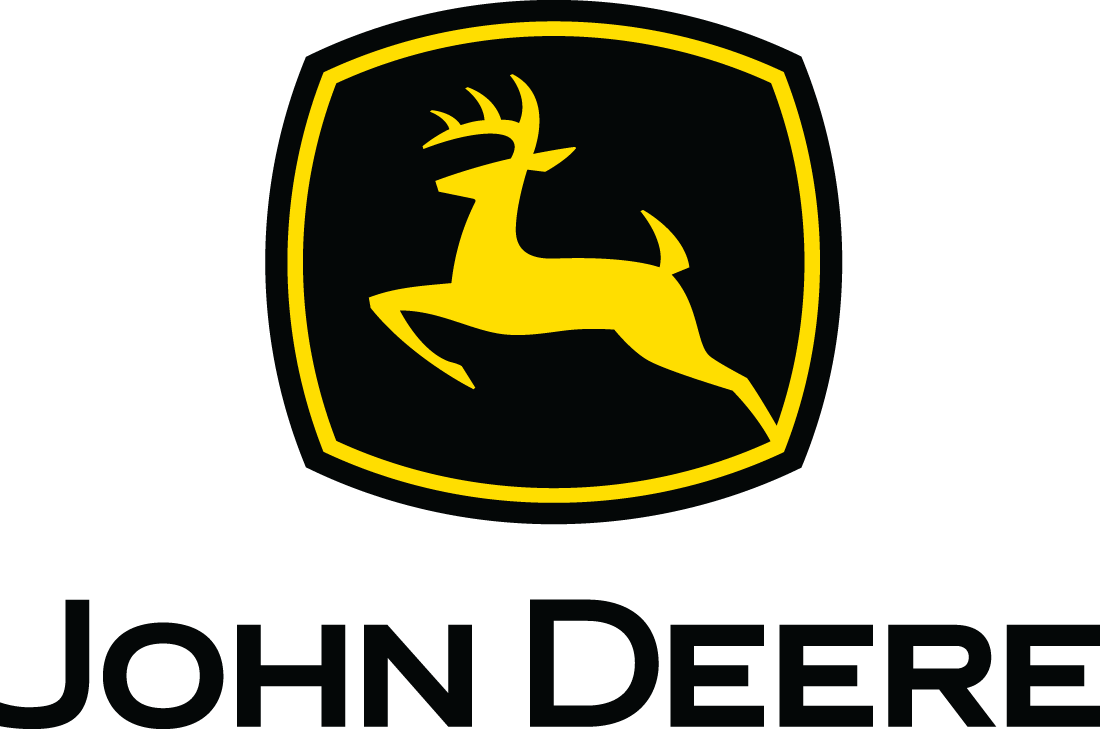
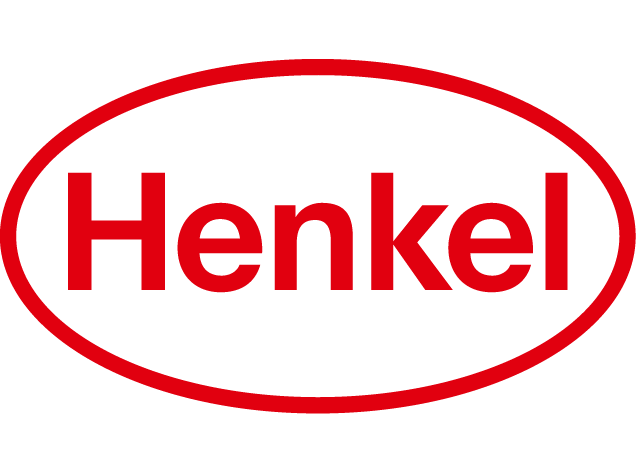
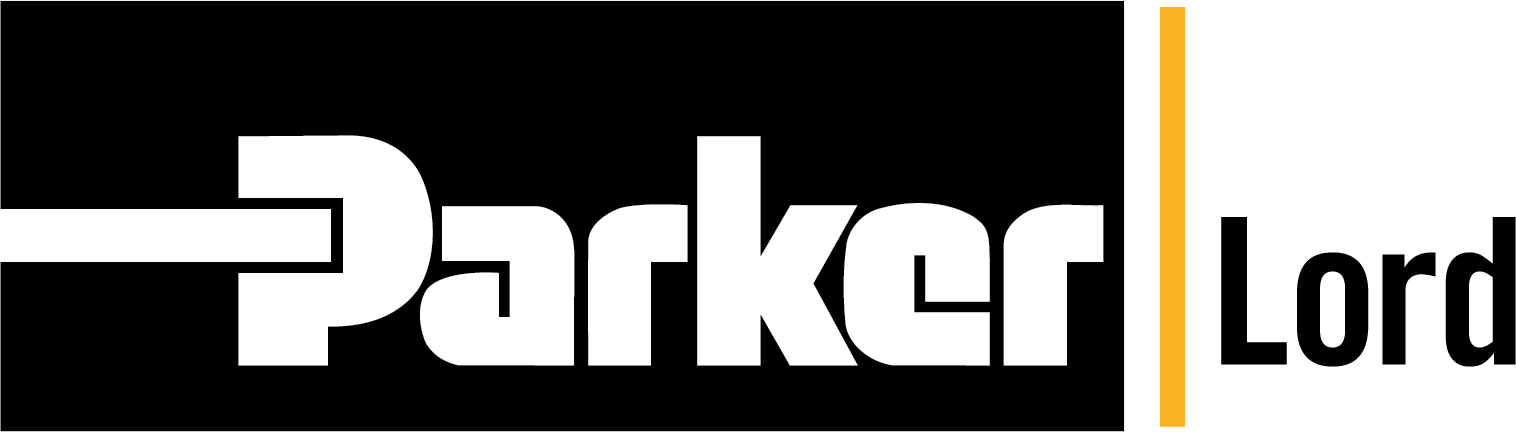
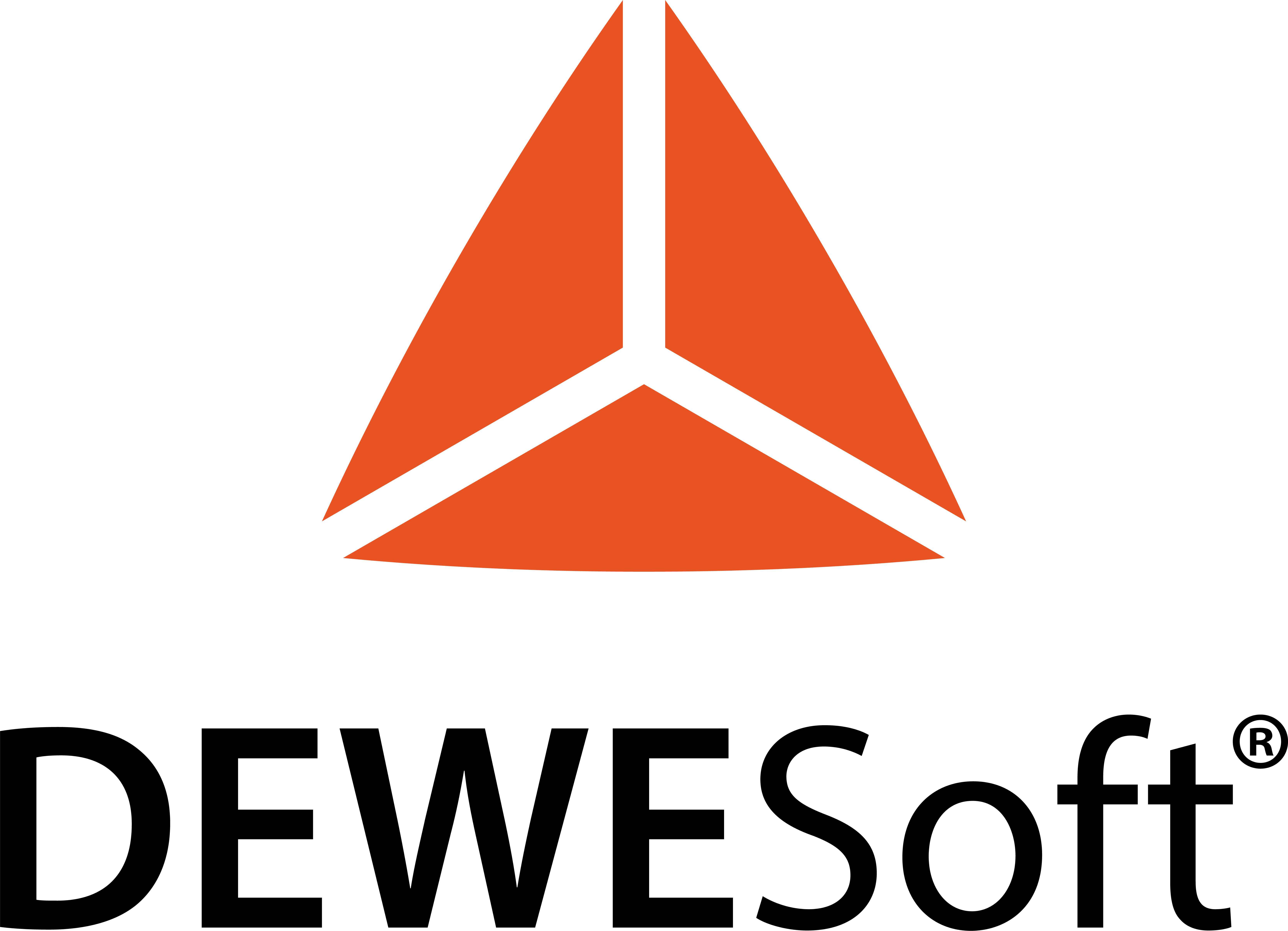
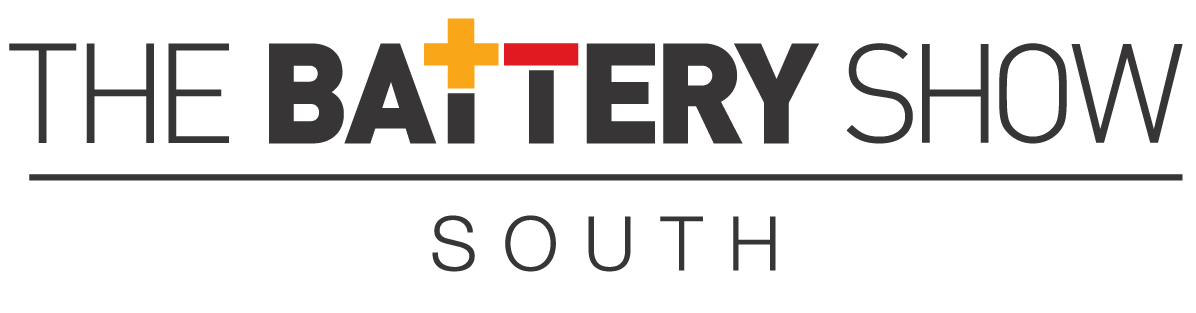
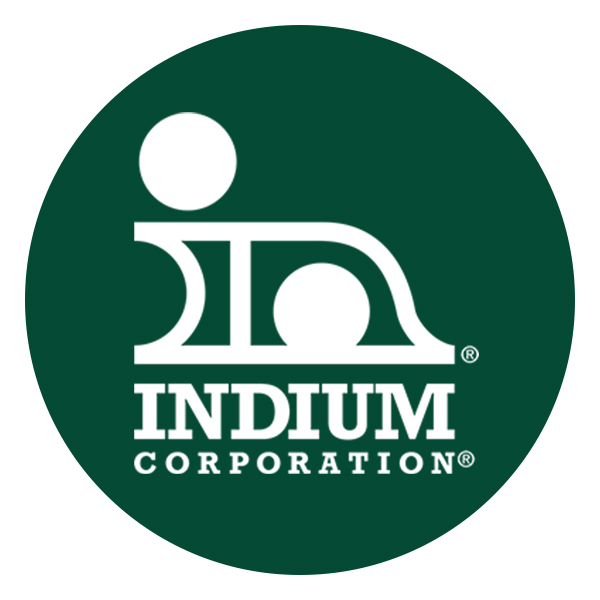

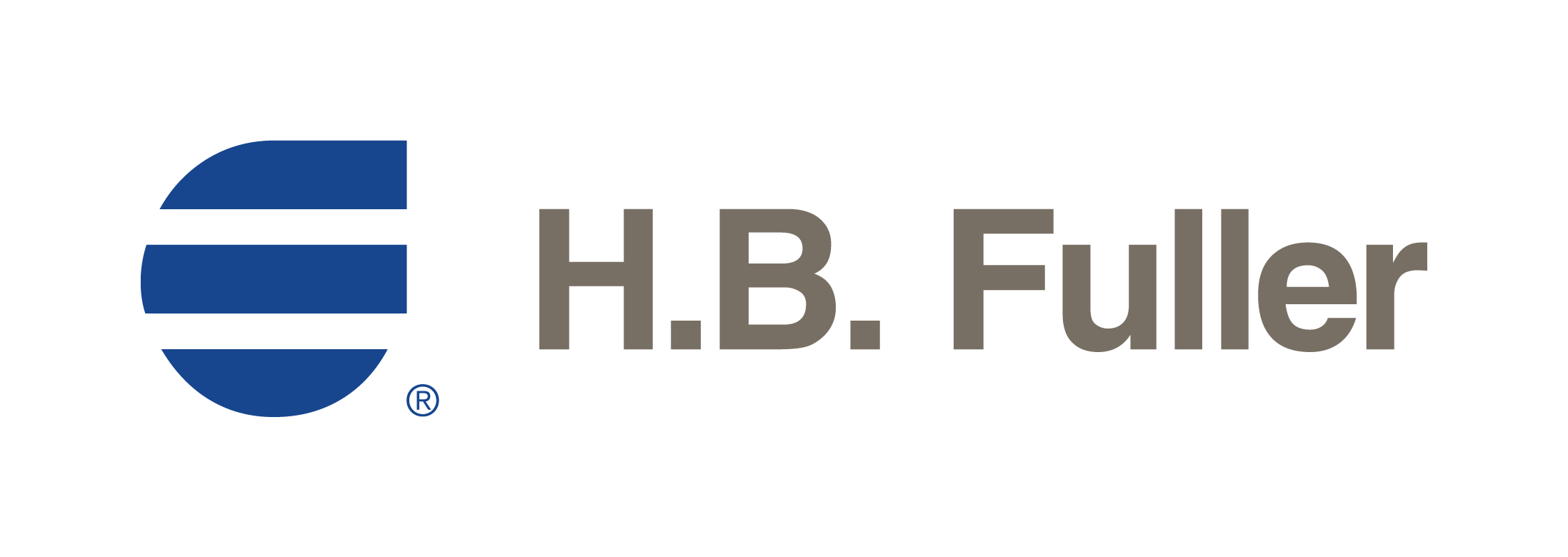
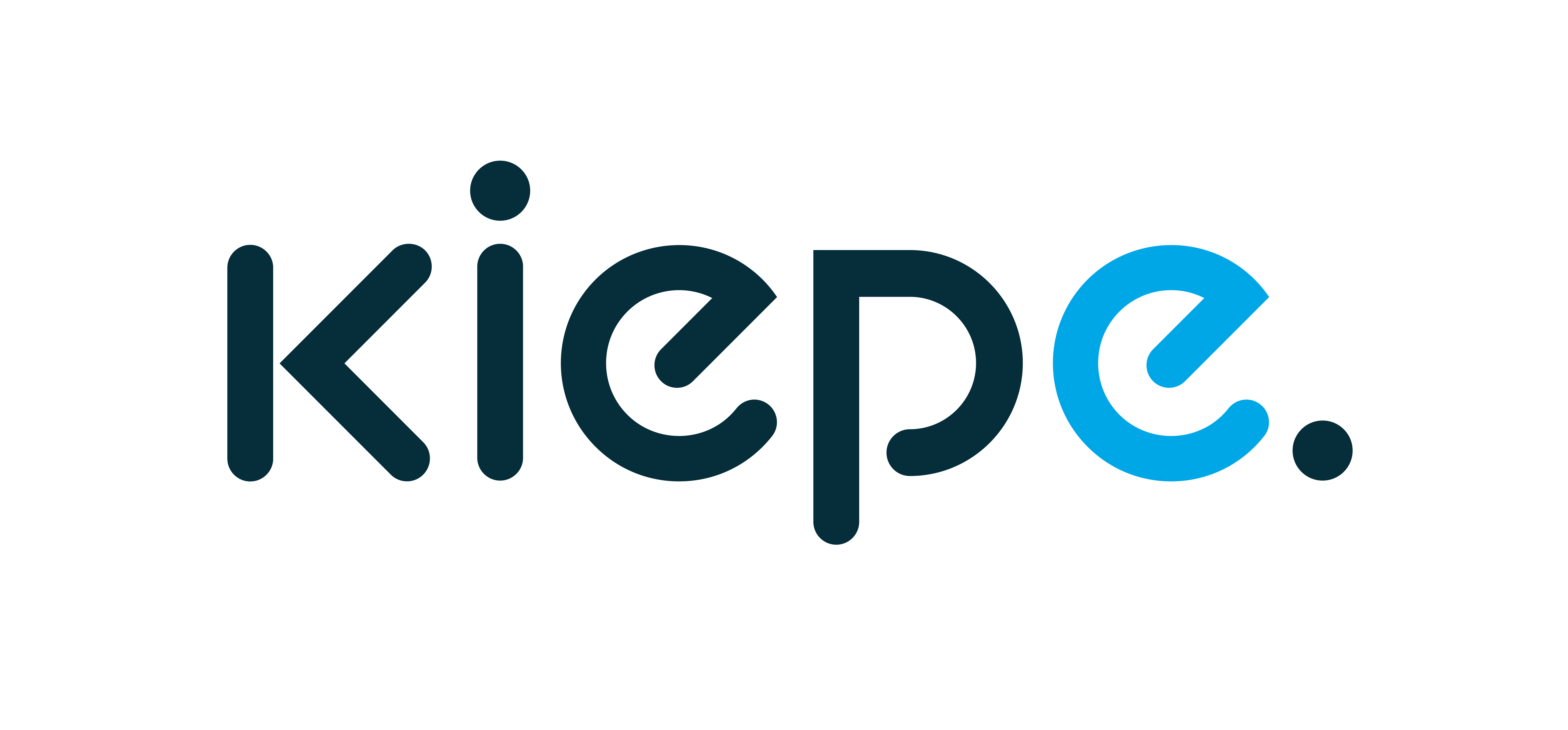
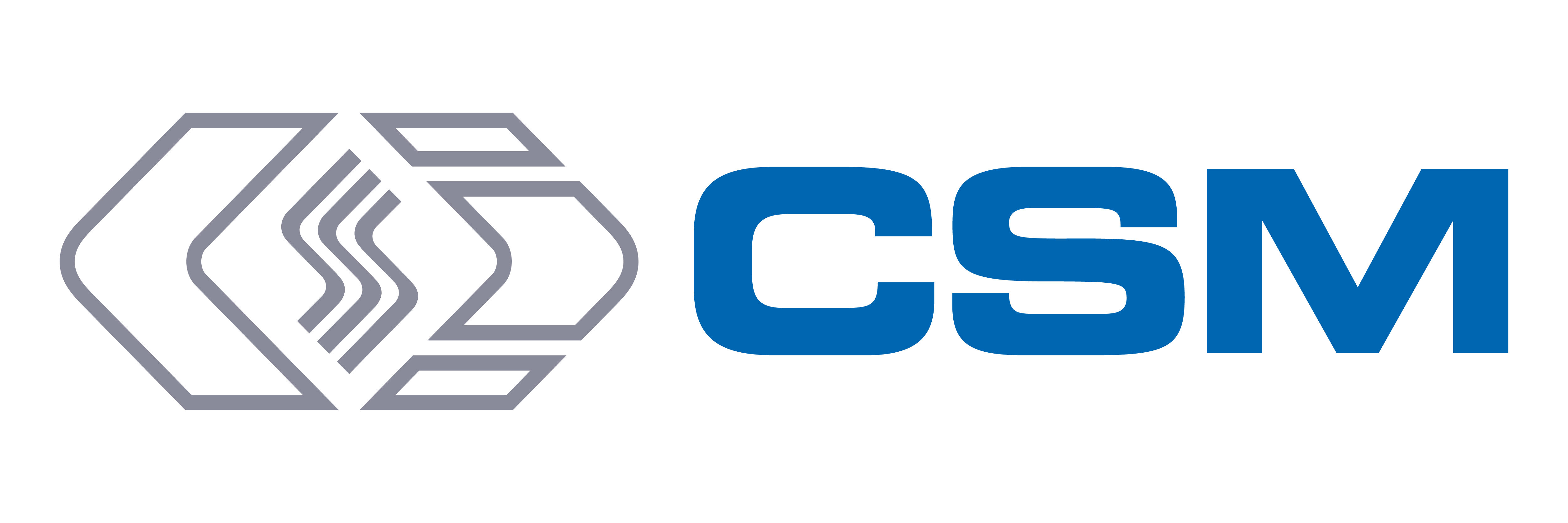