Cell ageing characteristics
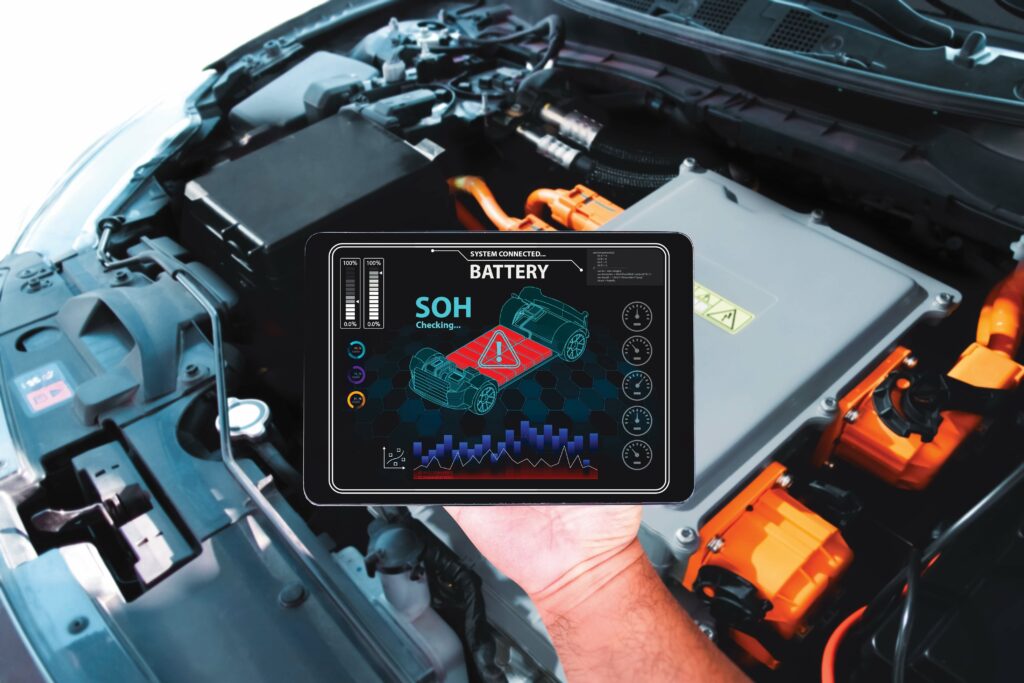
(Image courtesy of Geotab)
Offering a lifeline
Modelling battery cells at end of life is becoming more popular for key reasons, as Nick Flaherty reports
Accurate prediction of battery lifespan is crucial for e-mobility and involves a variety of test approaches, from thermal measurements to transmission electron microscopes. Assessing how a cell delivers power when required and how it is charged provides insights into its lifetime.
However, predicting battery lifespan accurately is challenging as the degradation in capacity is nonlinear, and it depends very much on the operating and charging conditions over the lifetime of the cell.
This is leading to an increasing focus on the modelling of battery cells at end of life to reliably squeeze out the maximum energy, reducing the total cost of ownership of a battery pack. Features such as dormant capacity are being identified, leading to new techniques to boost the amount of energy available from all kinds of cell chemistries.
Early models were developed by extensive testing of lithium-ion and lithium iron phosphate (LFP) cells with graphite anodes, and creating a model from that data, but performance can vary significantly between different batches of cells with the same chemistry on the same production line.
Many more battery chemistries are also becoming available, from silicon anodes and lithium metal cathodes to sulfur and sodium chemistries. There are also solid state battery structures with varying lifetime performances.
As a result, there is more interest in mathematical modelling of battery cells over their lifetime and analysis of patterns in the data. This is leading to the development of machine learning (ML) and deep-learning artificial intelligence (AI) models to evaluate and predict the performance of cells at end of life.
Based on data from over 6000 EVs, spanning all the major makes and models, Geotab found EV batteries are exhibiting high levels of sustained health. Across all vehicles, on average, an EV battery degrades at 1.8% per year, with the best performing at 1% per year. This compares with 2.3% in 2019.
“With these higher levels of sustained health, batteries in the latest EV models will comfortably outlast the usable life of the vehicle and will likely not need to be replaced,” says David Savage, vice-president for the UK and Ireland at Geotab.
“The fact is that a 1.8% decline in battery health is unlikely to have a significant impact on most drivers’ daily vehicle needs, and this number will only come down further with new EV models and improved battery technology.”
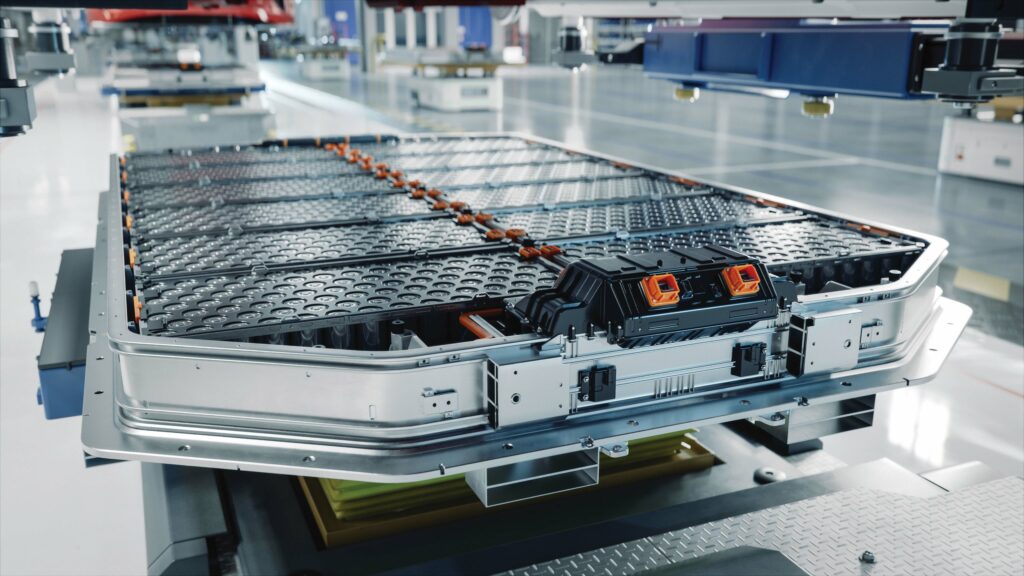
(Image courtesy of Geotab)
ML models
Developing AI models is particularly challenging under fast charging data. Extracting the data that these models need, called feature extraction, poses an additional challenge due to the large volume of experimental data, the unique conditions that each battery experiences and the degradation that occurs over its lifecycle.
Predicting the entire capacity degradation curve is a crucial indicator of battery state of health (SoH). Despite the inherent complexities, accurately predicting the evolution of the SoH carries significant value as it gives a better understanding of the battery’s future state, but also enables the formulation of more efficient charging protocols.
Researchers at the Hong Kong University of Science and Technology have developed a model that can automatically extract features from charge/discharge voltage profiles. This eliminates the need for preliminary
de-noising and pre-processing, such as differential voltage analysis, which is needed for other modelling techniques.
This uses AI techniques called Long Short-Term Memory (LSTM) and gated recurrent unit (GRU) neural networks to predict SoH and end-of-life. The average errors for end-of-life were as low as -1.27% when 80% of the cycle life was forecast.
Analysis of the whole discharge curve identified the most influential features, revealing that both the charge and discharge regions of voltage profiles serve as valuable predictors of battery ageing.
Recently, professor Chen Zhongwei and associate professor Mao Zhiyu from the Dalian Institute of Chemical Physics at the Chinese Academy of Sciences, in collaboration with professor Feng Jiangtao from Xi’an Jiaotong University, designed a new type of deep-learning model to predict the current cycle life (CCL) and remaining useful life (RUL) of the target battery.
The dual stream-vision transformer with efficient self-attention mechanism (DS-ViT-ESA) model was developed using a small amount of charging cycle data. It employs a different type of AI model, called a vision transformer, with a dual-stream framework to capture and integrate hidden features across multiple timescales.
The model was able to accurately predict the battery’s CCL and RUL. With just 15 charging cycle data points, it achieved RUL and CLL prediction errors of only 5.40% and 4.64%, respectively. Low prediction errors were maintained even when testing charging strategies not included in the training dataset, demonstrating its zero-shot generalisation capability.
This battery lifespan prediction model was also a crucial component of the first-generation Battery Digital Brain, called PBSRD Digit. The system integrated with this algorithm has significantly improved accuracy for EVs and can be deployed on either cloud servers or on the vehicle as part of the battery management system (BMS).
“The battery lifespan prediction model effectively balances prediction accuracy with computational cost, thereby increasing the application value of the Battery Digital Brain for lifespan estimation,” says Chen.
“We plan to further optimise the model using techniques such as model distillation and pruning, aiming to enhance robustness and resource utilisation of the system.”
This looks at the differences between each cycle as the supplementary model input, extracting the inner-cycle and cycle-to-cycle ageing data, which is then fused by a dual-stream structure to enhance prediction accuracy.
Lithium metal
Meanwhile, researchers in Japan have developed a model that can predict the cycle lives of high energy-density lithium-metal batteries. These have the potential to achieve higher energy densities per unit mass than those of the lithium-ion batteries currently in use for EVs.
The team at the National Institute for Materials Science (NIMS) worked with Japanese tech firm Softbank to apply ML methods to battery performance data. The model proved able to accurately estimate the lifetime of the battery cell by analysing the charge, discharge and voltage relaxation process data without relying on any assumptions about specific battery degradation mechanisms.
A lithium-metal battery with an energy density higher than 300 Wh/kg
and a life of more than 200 charge/discharge cycles has been developed, but needs accurate estimates of cycle life. However, degradation mechanisms are more complex in lithium-metal than conventional lithium-ion batteries and are not yet fully understood, making the development of models capable of predicting the cycle lives of the former a great challenge.
The research team built a large number of high energy-density lithium-metal battery cells, each composed of a lithium-metal anode and a nickel-rich cathode, and evaluated their charge/discharge performance.
They then constructed a model able to predict the cycle lives of lithium-metal batteries by applying ML methods to the charge/discharge data.
The model proved able to make accurate predictions by analysing charge, discharge and voltage-relaxation process data without relying on any assumptions about specific battery degradation mechanisms.
This will be used to improve the cycle life prediction accuracy of the model and speed up the development of high energy-density lithium-metal batteries.
Inaccurate SoC estimations can lead to overcharging, faster degradation, and early battery disposal. To address this, researchers at Canterbury Christ Church University in the UK developed an AI model to accurately estimate the State of Charge by analysing real-time data to predict battery health levels more precisely than traditional methods.
The Cluster-Based Learning Model (CBLM) can enhance BMS designs, allowing for more precise monitoring and preventing overcharging or discharging at end of life.
“This research is not just about making batteries last longer; it’s about making them smarter, safer and more reliable. By improving SoC estimation, we can extend the life of second-life batteries, reduce waste, and make energy storage systems more efficient and cost-effective, and in turn reduce our reliance on fossil fuels,” said Dr Hany Hassanin, reader in engineering at the university.
“The research team are keen to collaborate with industry partners to bring this technology from the lab to real-world applications.”
The CBLM model was tested on Tesla Model lithium-ion batteries and achieved lower estimation errors than state-of-the-art methods. It preserved 5.4% more battery capacity, slowing degradation and extending operational life, and it is also 60% faster than existing methods.
Property | Molicel P45B | Molicel P50B | Comment |
Capacity (Ah) | 4.5 | 5 | 11% higher |
Mass (g) | 66 | 68.8 | 4% higher |
Specific energy (Wh/kg) | 245 | 260 | 7% higher |
Energy density (Wh/L) | 643 | 714 | 11% higher |
Peak current (A) | 13.5 (charge) / 45 (discharge) | 15 (charge) / 50 (discharge) | 11% higher |
DCIR at 50% (MΩ) | 15 | 15 | No change |
(Image courtesy of About:Energy)
Model comparison
When evaluating battery technologies, the process is technically and commercially demanding. Beyond engineering metrics like energy density, factors such as supplier reliability and production capacity play a key role in choosing the right cell for a vehicle programme. High energy density doesn’t always equal better performance; the power-to-energy ratio required for the platform may vary or the battery life could be limiting.
One analysis of the latest Molicel P50B high-performance lithium-ion cell with a silicon anode assessed a batch of low-volume, production-run cells that had undergone a rigorous testing process.
Compared with the previous P45B cell, the P50B has higher capacity, faster charging and enhanced discharge capabilities, all while maintaining the same level of resistance.
Although the P50B is slightly heavier, its increased energy density suggests a higher silicon content and more optimisation of the jelly-roll to boost total cell capacity. This can create a lighter pack or extend range and lifespan. The different internal chemistry introduces new performance characteristics and potential challenges.
For instance, the increased silicon in the negative electrode may alter degradation patterns, affecting long-term reliability and complicating battery state estimation due to greater hysteresis. To benefit fully from the P50B, understanding these nuances is essential; hence the need for the model over the lifetime of the cell.
For a baseline battery pack using the P45B in a 240s 8p configuration, this would deliver about 186 Wh/kg at pack level, resulting in a total energy capacity of about 31.5 kWh for a 169 kg pack.
Using the P50B cells while maintaining the same 169 kg pack achieves the same 31.5 kWh output by cycling only about 90% of the SoC window, compared with the full 100% SoC required for the P45B.
A reduced SoC window of operation would be expected to extend battery life and reduce ageing. Alternatively, using the full SoC window with the P50B cells could deliver nearly 200 Wh/kg and an overall energy capacity of 35 kWh.
The higher capacity of the P50B would allow for lower operating currents, which would reduce the thermal load on the BMS, and again extend the lifetime and reduce ageing by reducing the stress on each cell. On average, an individual P50B cell would carry 11% less current than an individual P50B cell for the same pack-level current demand.
The electrical models with lumped thermal feedback are used to evaluate the differences between the P45B and P50B. These models have been parameterised and validated using a cell measurement and modelling approach that achieves a root-mean-squared error (RMSE) of less than 30 mV in simulated voltage across the cells’ standard operating envelopes.
This low margin of error enables reliable estimation of key parameters such as available power, SoC, heat generation rates and cell temperature rise.
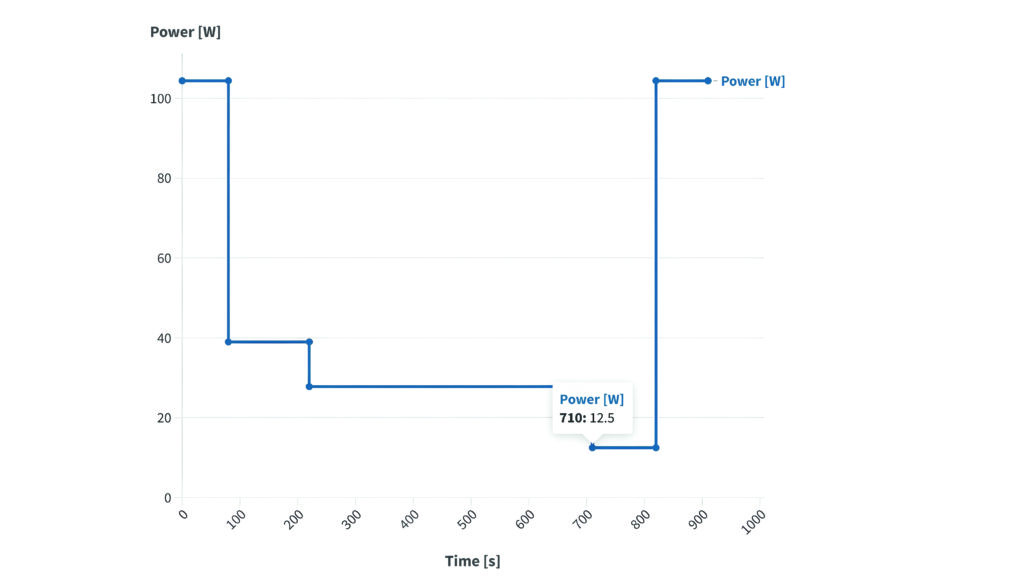
(Image courtesy of About:Energy)
Mission profile
The higher energy density of the P50B is popular with eVTOL air-taxi designs, so simulations focus on a custom eVTOL flight profile to show how long the cells will last in a specific application. This profile includes typical mission phases, such as takeoff, landing, transition and cruise.
The simulations were initiated with a SoC of 100% and a starting temperature of 25 C, with a heat sink of 0.5 W per cell to simulate the thermal-management system typically used in eVTOLs.
During the take-off phase of the eVTOL mission profile, the P45B initially exhibits a smaller voltage drop compared with the P50B because of the faster heat buildup.
As the flight progresses, the P50B’s higher capacity comes into play, allowing it to maintain a higher overall voltage. This highlights how the P50B’s improved performance is largely tied to its thermal characteristics, which can have significant implications for pack design and longevity.
In practice, at an individual cell level, the P50B would potentially see a lower current demand due to higher pack-level capacity than the P45B.
Metric | Molicel P45B | Molicel P50B |
Final SoC (%) | 35.4 | 42.2 |
Minimum voltage (V) | 3.185 | 3.242 |
Max temperature (C) | 59.8 | 57.7 |
(Image courtesy of About:Energy)
While the P50B outperformed the P45B in terms of final SoC and minimum voltage, it also generated less heat and experienced a lower temperature rise, primarily due to the lower operating currents in the P50B, despite similar resistance values and overpotentials between the two cells.
This suggests that one of the most immediate benefits of the P50B over the P45B may be the simplification of thermal-management system design. This gives more flexibility, such as reallocating weight budgets to enable a larger pack or offering an extended warranty, and it should improve cell-to-cell consistency, enhance pack performance and extend battery life.
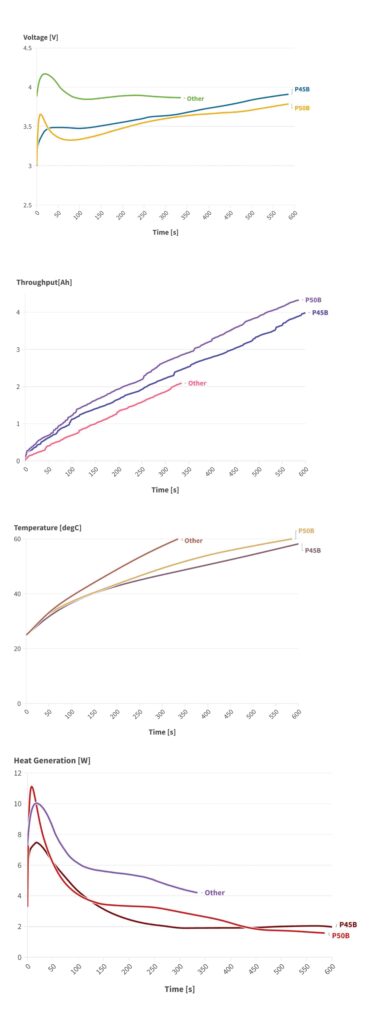
(Image courtesy of About:Energy)
Fast-charge performance
The charging performance of cells is also key for modelling. The P45B and P50B cells were compared with a typical 5 Ah, 21700 lithium-ion cell with an NMC cathode electrode rather than silicon. The cells were charged from 5% SoC using a constant 50 W power. If any cell hit 4.2 V, it switched to constant voltage (CV) mode.
The aim was to either maintain a 50 W charge for 10 minutes or measure the charge capacity before reaching 60 C. All tests started at 25 C with no active cooling, and the results were based on simulations using electrical-equivalent circuit models (ECMs) with thermal feedback.
Both the Molicel P45B and P50B cells far outperformed the generic 21700 cell, which couldn’t complete the 10-minute charge cycle, reaching 60 C and 4.2 V in about five minutes, leading to premature termination due to excessive heat.
In contrast, the Molicel cells managed heat effectively and maintained better charging efficiency as a result of the lower internal resistance, which reduces heat generation. It also helps to avoid negative potentials that can harm battery performance and lifespan. Lower internal resistance contributes to more stable operation and less thermal stress, which can significantly extend battery life and enhance safety.
2D thermal models
A 2D thermal model is a key way of investigating the temperature distribution across the P45B and the P50B. To ensure both cells had a fair comparison, the eVTOL heat-generation profile was used.
The thermal model varies from the electrical model due to the different ways in which thermal phenomena are represented: the electrical model integrates thermal behaviour with electrical performance, while the 2D thermal model uses a common heat-generation profile.
As the cells are cooled from the base, the temperatures at the cap are much higher than at the base, which would be closer to the coolant temperature. This is crucial to optimise, because while a greater cooling magnitude may drop the bulk temperature of the cell, parts of the cell would see a much higher temperature, leading to premature degradation and safety issues.
Even small variations in temperature within a battery cell can lead to accelerated degradation and reduced performance as it ages. Advanced thermal-modelling techniques are essential as they offer precise predictions of temperature behaviour and degradation patterns. These insights enable the development of improved design and management strategies for battery systems, enhancing their reliability and efficiency.
A 2D thermal model provides detailed temperature distribution data, whether this is used to refine initial estimates from electrical models or in combination with them.
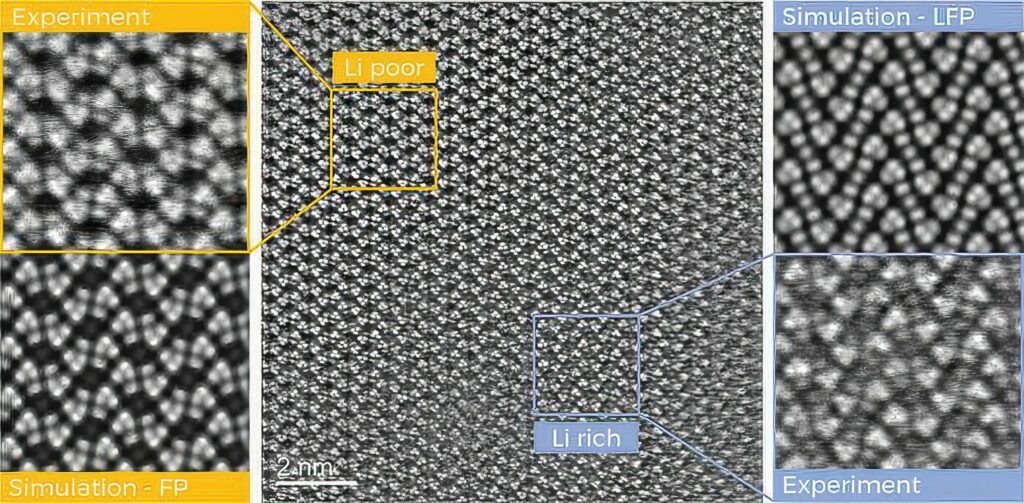
(Image courtesy of TU Graz)
Degradation testing
While manufacturer data and basic estimates offer initial insights, detailed design decisions require advanced degradation testing. Tests on both the P45B and P50B cells using a temperature-controlled test fixture involved aggressive charge and discharge cycles (constant power) at a typical operating temperature that is relevant for high-power, energy-dense and weight-sensitive cells.
Assuming the end-of-life capacity for both the P45B and P50B is defined as 80% of the initial capacity (i.e. 3.6 Ah), the tests show the P50B lasts up to 50% longer in terms of cycle life compared with the P45B before reaching this 3.6 Ah threshold.
This is based on 0.1 C-Rate discharge capacity checks conducted every 50 cycles. Additionally, direct current internal resistance (DCIR) testing at 50% SoC, conducted every 50 cycles, showed the P50B’s relative resistance increased at a slower rate than the P45B. This is significant, not only in terms of the available energy from the cells, but also for potential differences in thermal-management requirements at pack level.
This shows that while the P50B offers the potential to enhance either performance or lifespan as a direct replacement, achieving both simultaneously may require adjustments to pack-level operations.
For example, operating the P50B within a narrower SoC window could help mitigate the silicon-related degradation that becomes more pronounced at lower SoC values.
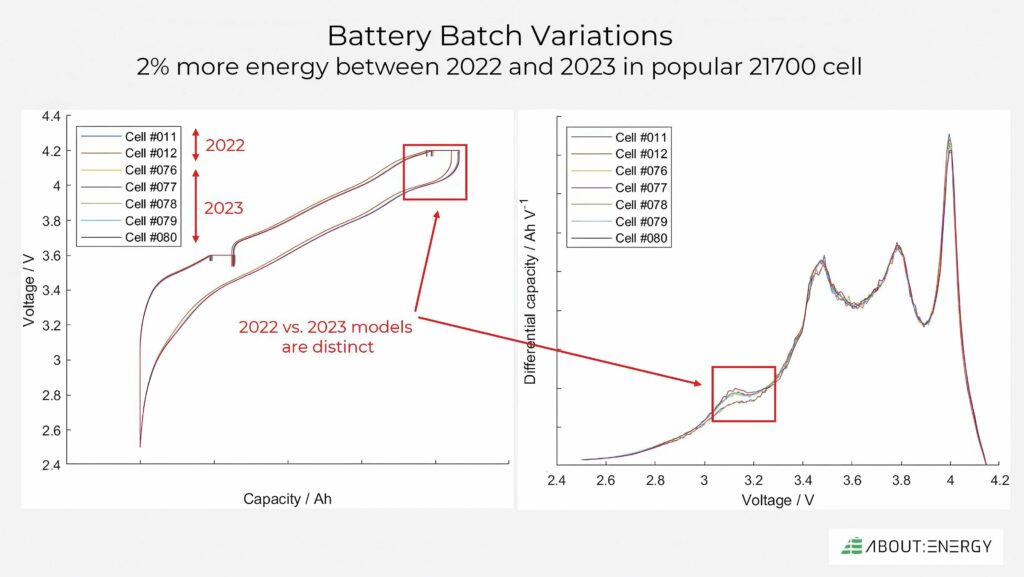
(Image courtesy of About:Energy)
Dormant capacity
Lithium iron phosphate is one of the most important materials for batteries in electric cars, stationary energy storage systems and tools. It has a long service life, is comparatively inexpensive and does not tend to catch fire. However, there are still questions as to why lithium iron phosphate batteries do not reach the theoretical electricity storage capacity, sometimes by up to 25%.
Researchers at Graz University of Technology (TU Graz) in Austria have been exploring what happens to the lithium ions at end of life to create this dormant capacity. They used a transmission electron microscope (TEM) to track the lithium ions as they travelled through the battery material, map the arrangement in the crystal lattice of an iron phosphate cathode with unprecedented resolution, and precisely quantify the distribution in the crystal.
“Our investigations have shown that even when the test battery cells are fully charged, lithium ions remain in the crystal lattice of the cathode instead of migrating to the anode. These immobile ions incur a cost in capacity,” says Daniel Knez of the Institute of Electron Microscopy and Nanoanalysis at TU Graz.
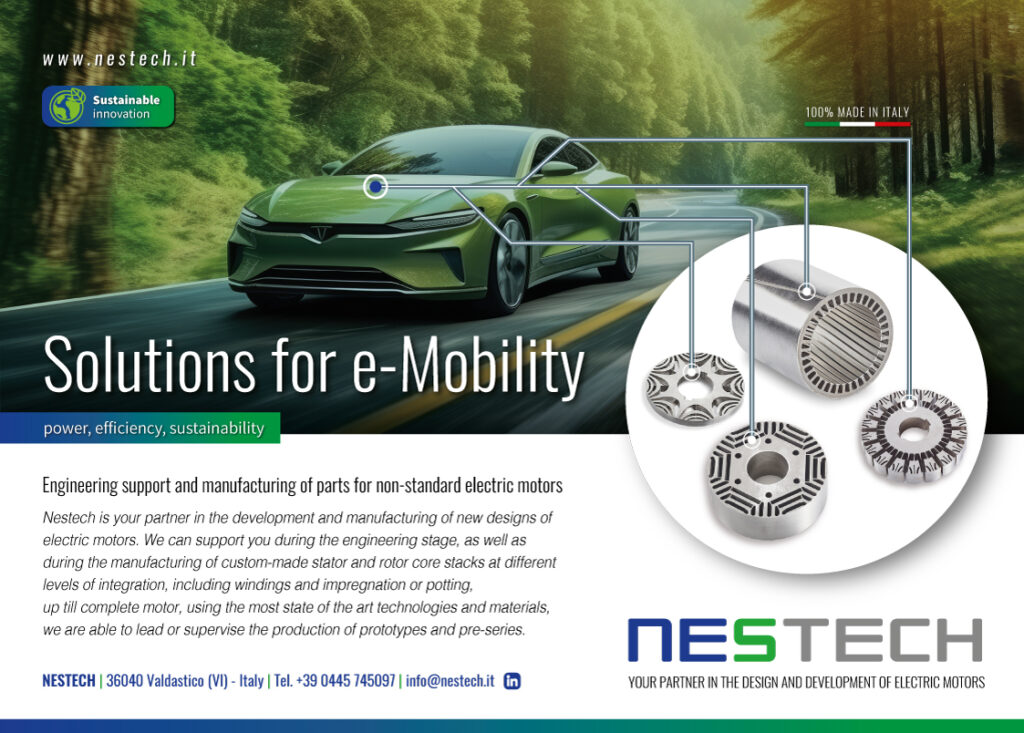
The immobile lithium ions are unevenly distributed in the cathode, but the team succeeded in precisely determining these areas of different levels of lithium enrichment and separating them from each other, down to a few nanometres. Distortions and deformations were found in the crystal lattice of the cathode in the transition areas.
“These details provide important information on physical effects that have so far counteracted battery efficiency, which we can take into account in the development of the materials,” says Ilie Hanzu of the Institute for Chemistry and Technology of Materials at TU Graz.
Samples of the electrodes of charged and discharged LFP batteries were analysed with the atomic-resolution ASTEM microscope at TU Graz. They combined electron energy loss spectroscopy with electron diffraction measurements and atomic-level imaging.
“By combining different examination methods, we were able to determine where the lithium is positioned in the crystal channels and how it gets there,” says researcher Nikola Šimić at the Institute of Electron Microscopy and Nanoanalysis.
“The methods we have developed and the knowledge we have gained about ion diffusion can be transferred to other battery materials with only minor adjustments in order to characterise them even more precisely, and develop them further.”
End-of-life boost
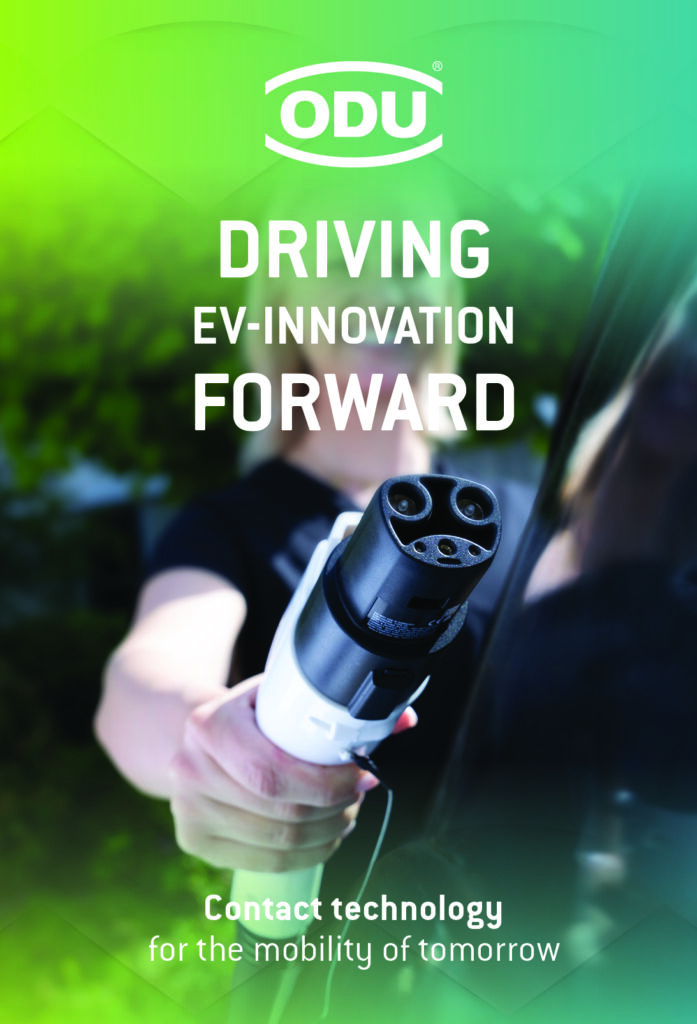
This capacity loss attributed to isolated active materials remains a challenge, so researchers in the US have developed an approach to substantially recover them in silicon electrodes. They used a voltage pulse to reconnect the isolated lithium-silicon particles back to the conductive network.
As a battery’s capacity diminishes over time, so does its ability to store and deliver power. Repeated cycles of charging and discharging cause a substantial volumetric change in the electrodes, which leads to severe structural deformation.
This problem is worse for high-capacity electrode materials that store a large amount of energy, such as lithium and silicon. Active material constituents of such electrodes can detach during charging, losing electrical connection to the rest of the battery components.
Using a five-second, high-current pulse, the team from Stanford University achieved more than 30% of capacity recovery in both lithium-silicon and si-lithium iron phosphate (Si-LFP) batteries. The recovered capacity sustains and replicates through multiple pulses, giving a constant capacity advantage. This uses a phenomenon known as dielectrophoresis – the movement of neutral, isolated LiSi particles under a localised, non-uniform electric field.
Modelling batches
Being able to track changes in a product line is crucially important for designing battery packs, creating warranties, and even for certification if the performance changes have a material impact on key metrics such as energy and lifespan.
In one popular 21700 cell, there was a significant change between models in 2022 and 2023. This was identified from differential capacity analysis, with a change in a silicon process matched to a specific peak, with the change probably due to an increase in silicon or a formulation adjustment.
This requires more flexible modelling that can take into account the variations in production. Being able to do this from minimal test data is vital, and the models have to be as accurate as possible.
All of this focus on the end of life of a battery cell helps to improve the performance of the platform via the BMS. The enhanced control of charging and discharging cycles from these models provides the power required without damaging the cells, allowing battery packs to last longer and improving the overall cost of ownership.
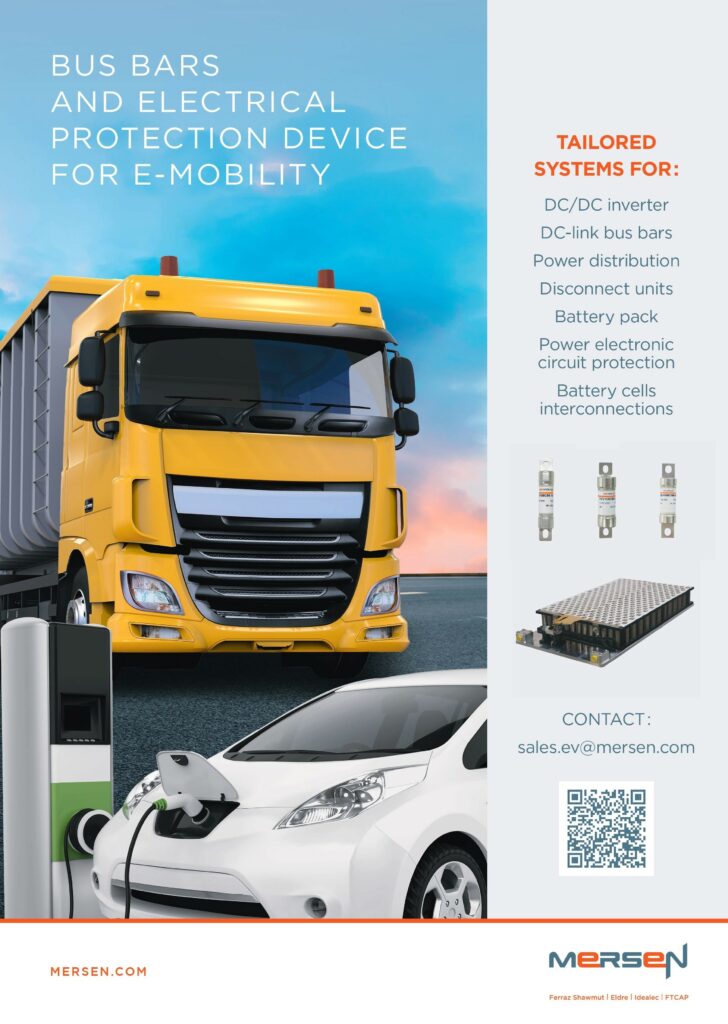
ONLINE PARTNERS
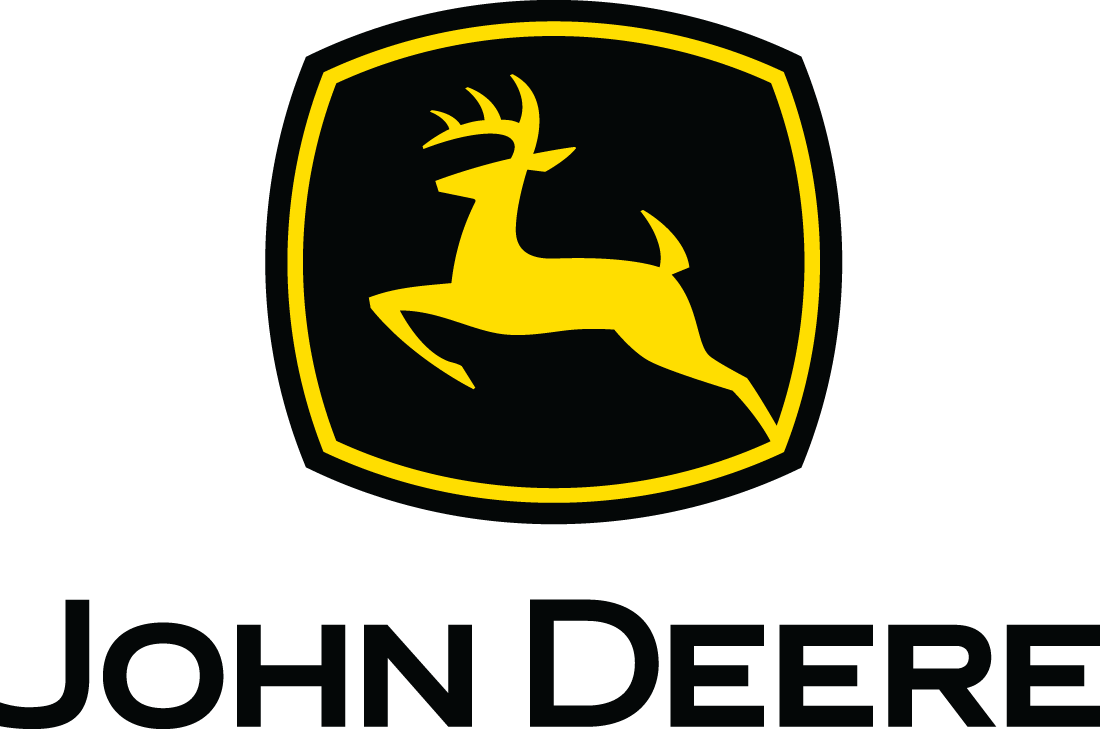
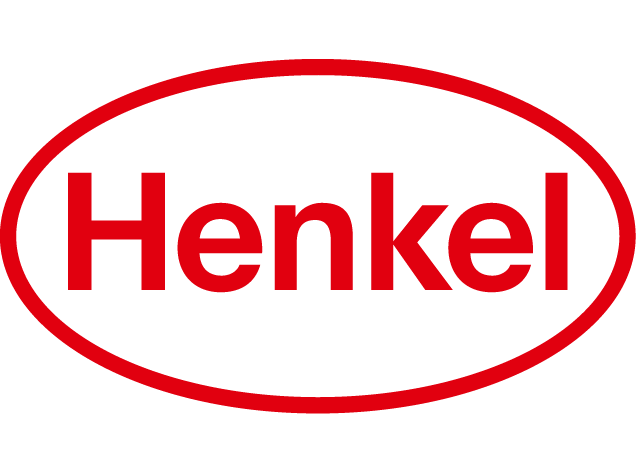
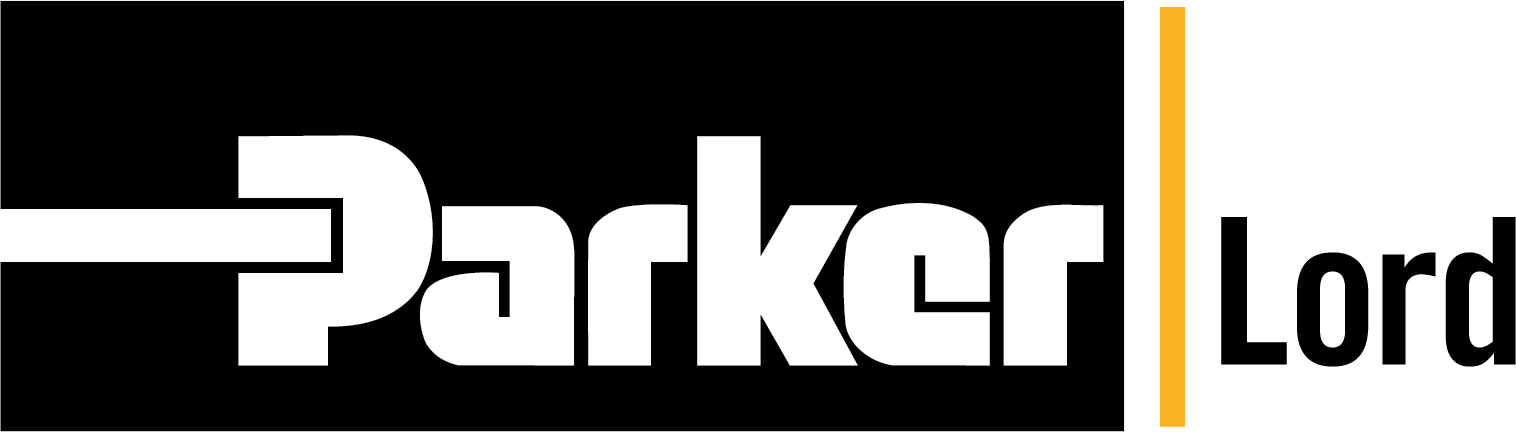
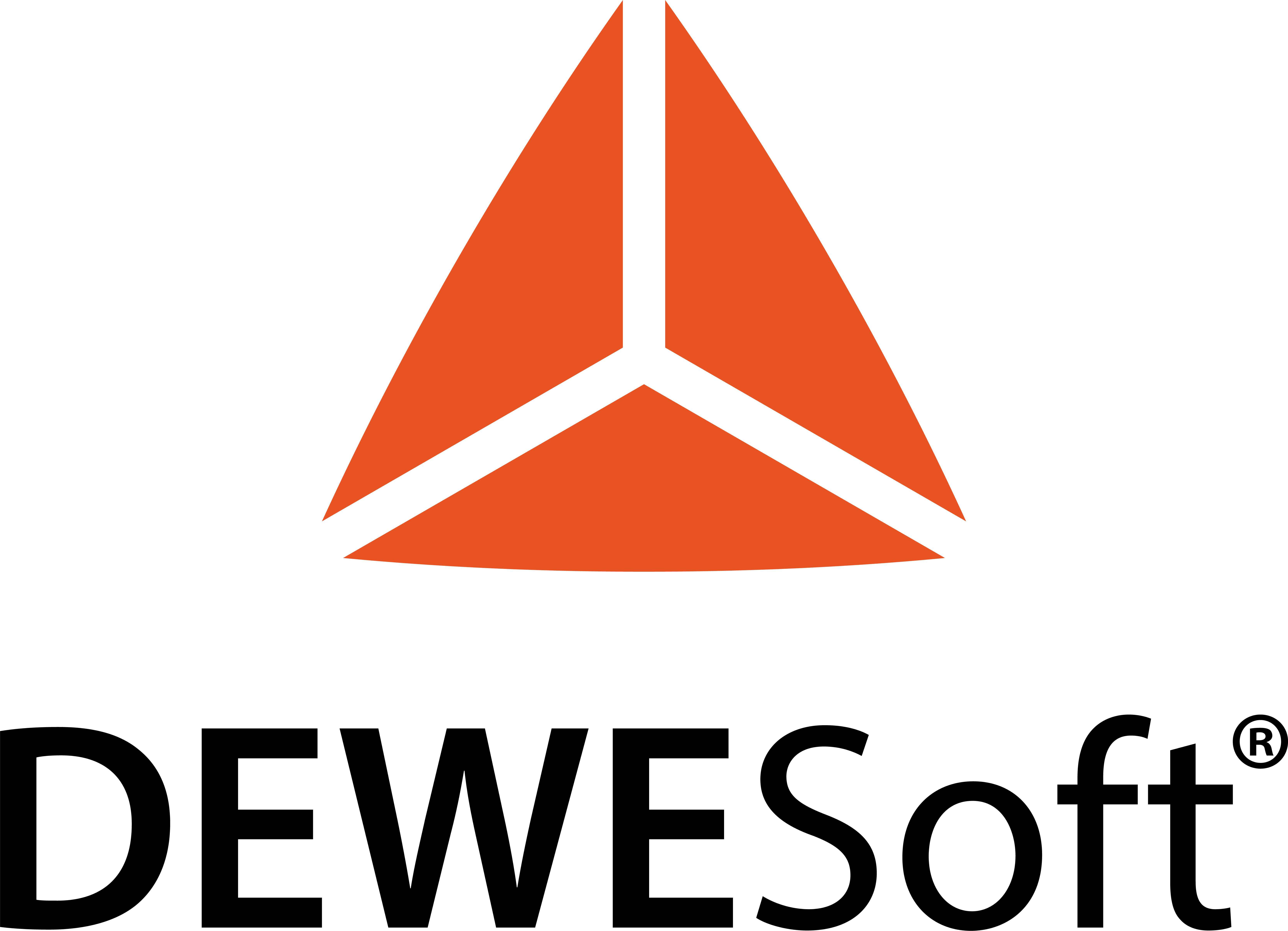
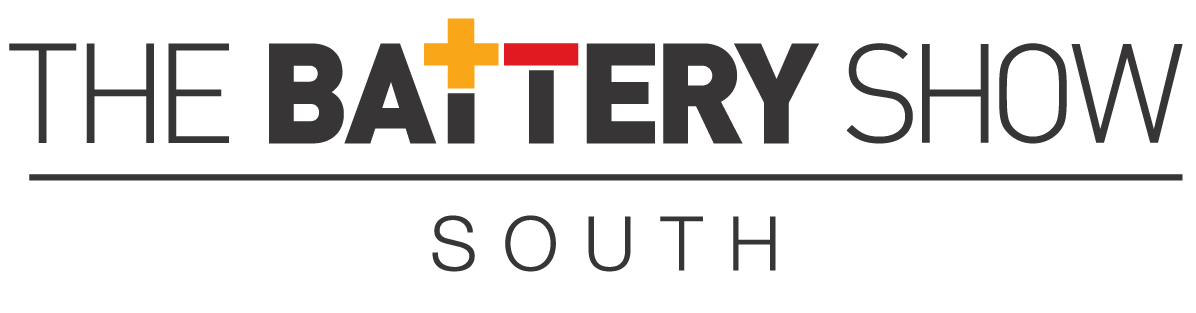
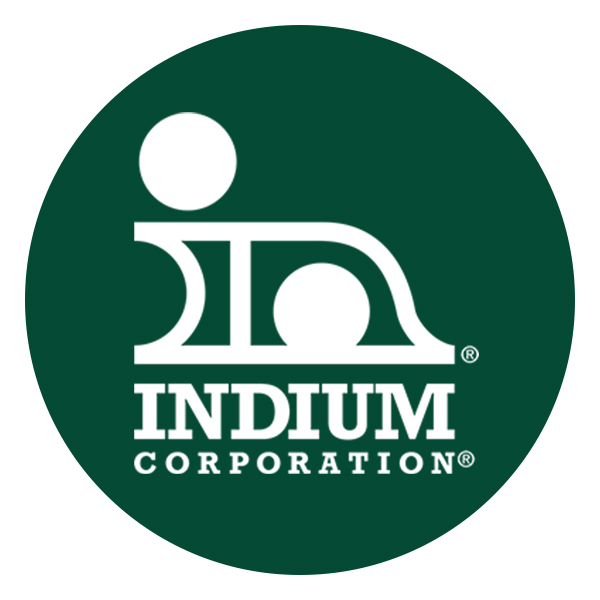

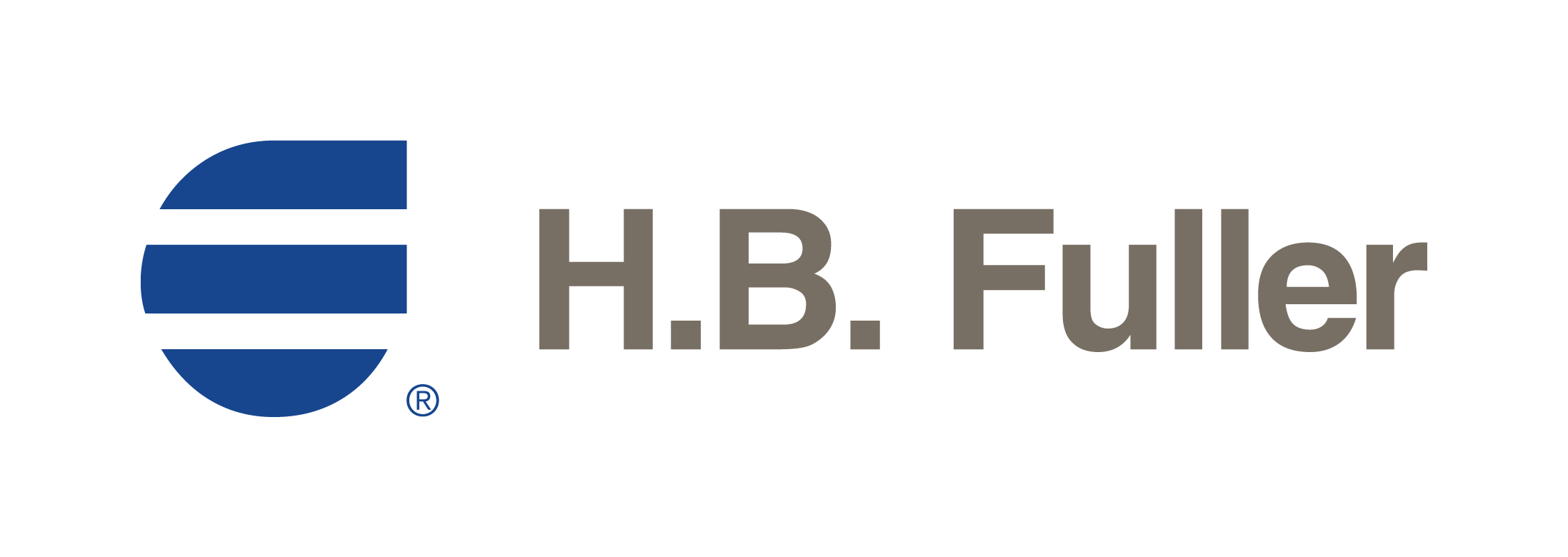
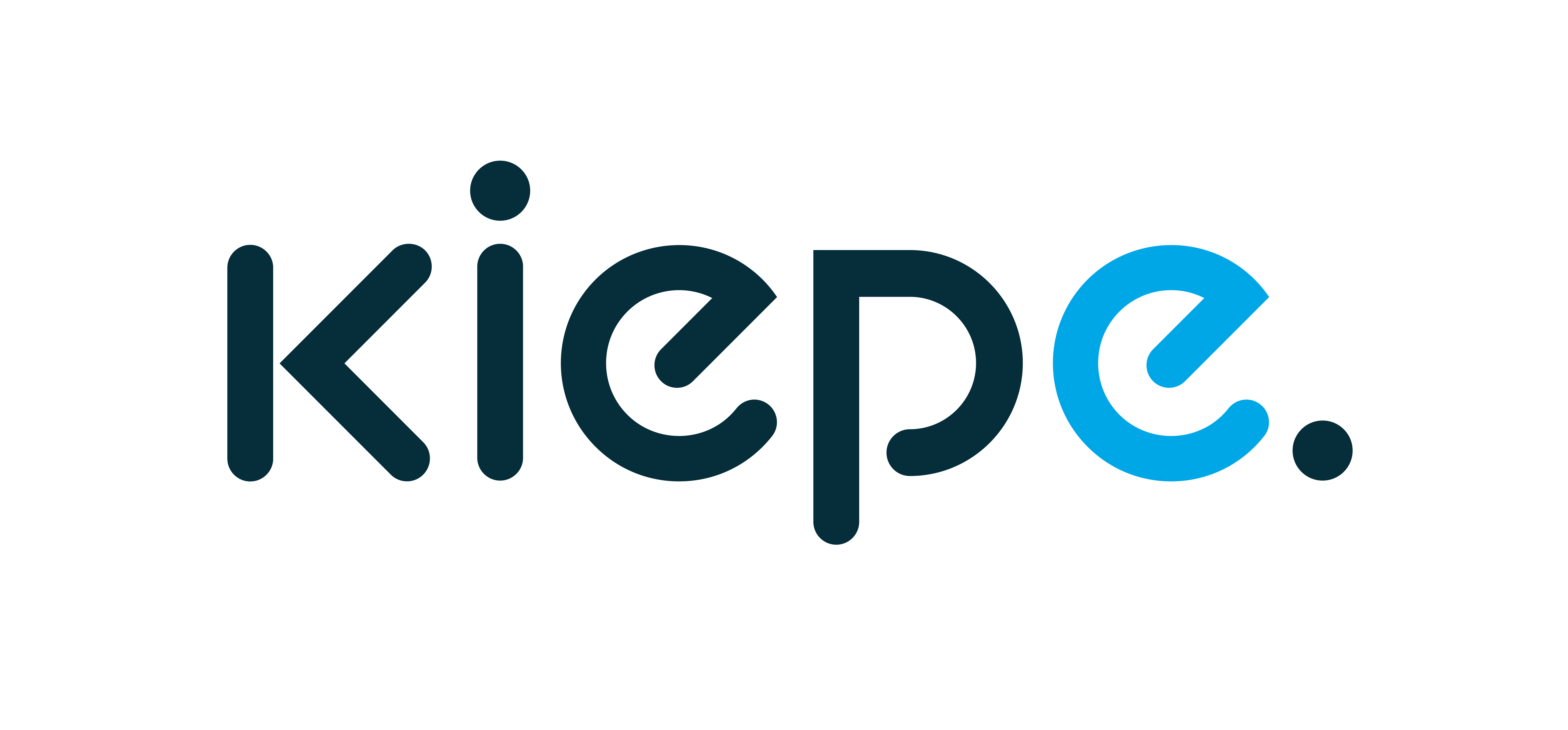
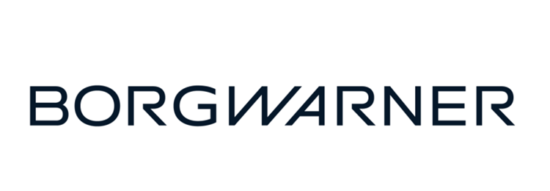